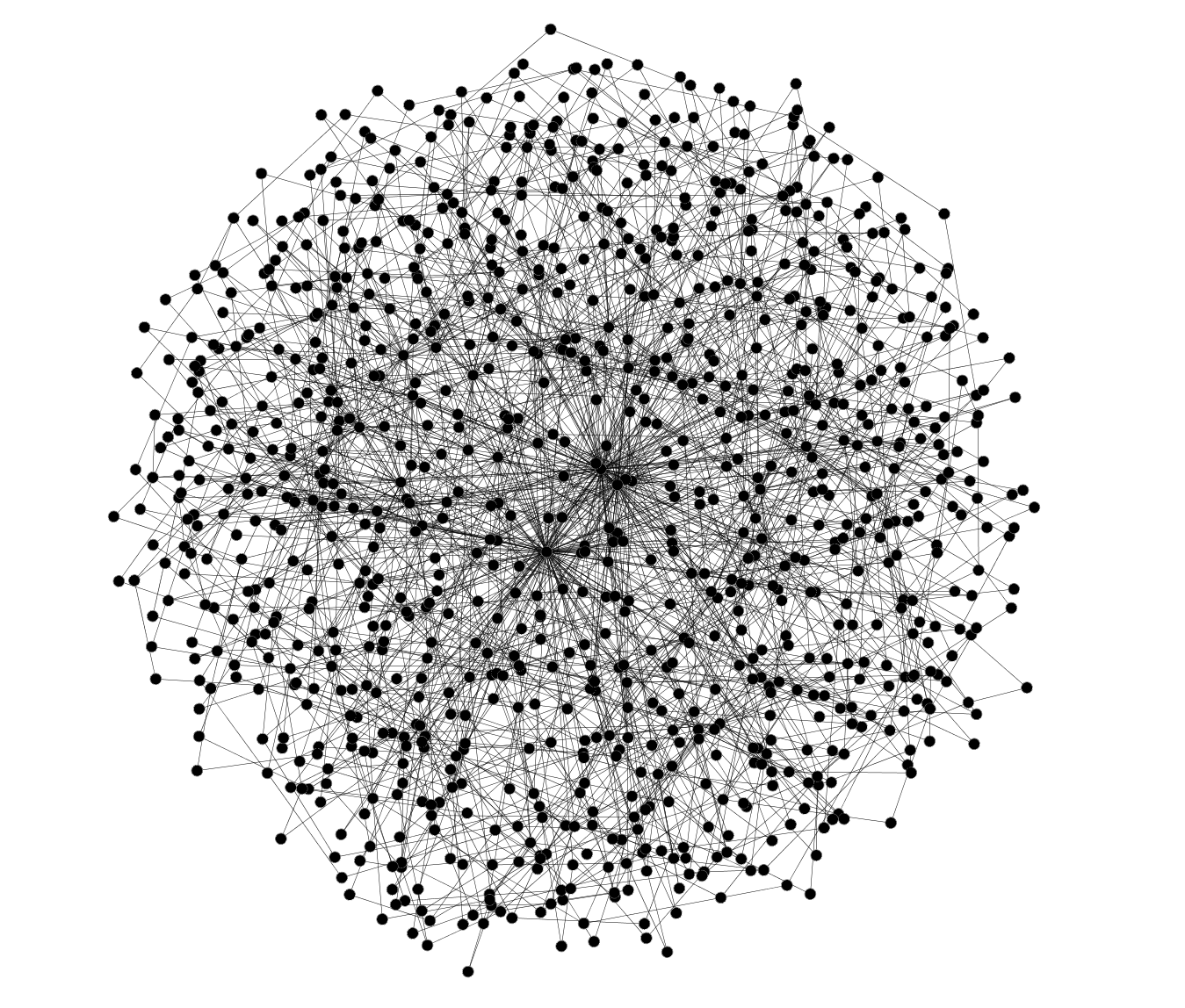
Opinion: Chance & Decision-Making
🎲 𝐂𝐡𝐚𝐧𝐜𝐞 & 📈 𝐃𝐞𝐜𝐢𝐬𝐢𝐨𝐧-𝐌𝐚𝐤𝐢𝐧𝐠 — Long before I called myself a data scientist, I helped build a backend website for sports betting on my very first job. After that, for about a decade, I improved the user experience for gambling sites of all kinds. As it turns out, the first data I engaged with professionally taught me a lot about human nature.
Throughout human history, we have been fascinated with chance. The first known tools used to this end were knucklebones in ancient Sumer, either for fortune-telling or games of chance. Better tools have been invented since, like dice, playing cards, and more recently, random number generators (RNGs). However, now we wield randomness for business/scientific purposes and not just mysticism/entertainment. In fact, the most powerful #MachineLearning methods depend on RNGs.
Above picture by: MET Museum (1 & 2) and World of Playing Cards, Featured image by: Simon Cockell
I recently read the book 𝘛𝘩𝘦 𝘋𝘳𝘶𝘯𝘬𝘢𝘳𝘥𝘴 𝘞𝘢𝘭𝘬, which made me reflect on what drew me to the discipline. We are surrounded by randomness, but humans want to be in control, often attributing skill to successful random events (ɢᴀᴍʙʟᴇʀ'ꜱ ꜰᴀʟʟᴀᴄʏ), and lack thereof otherwise. #Data can improve decisions by separating the signal from the noise and tracing outcomes to plausible causes. This possibility is what inspires my journey! What's yours?
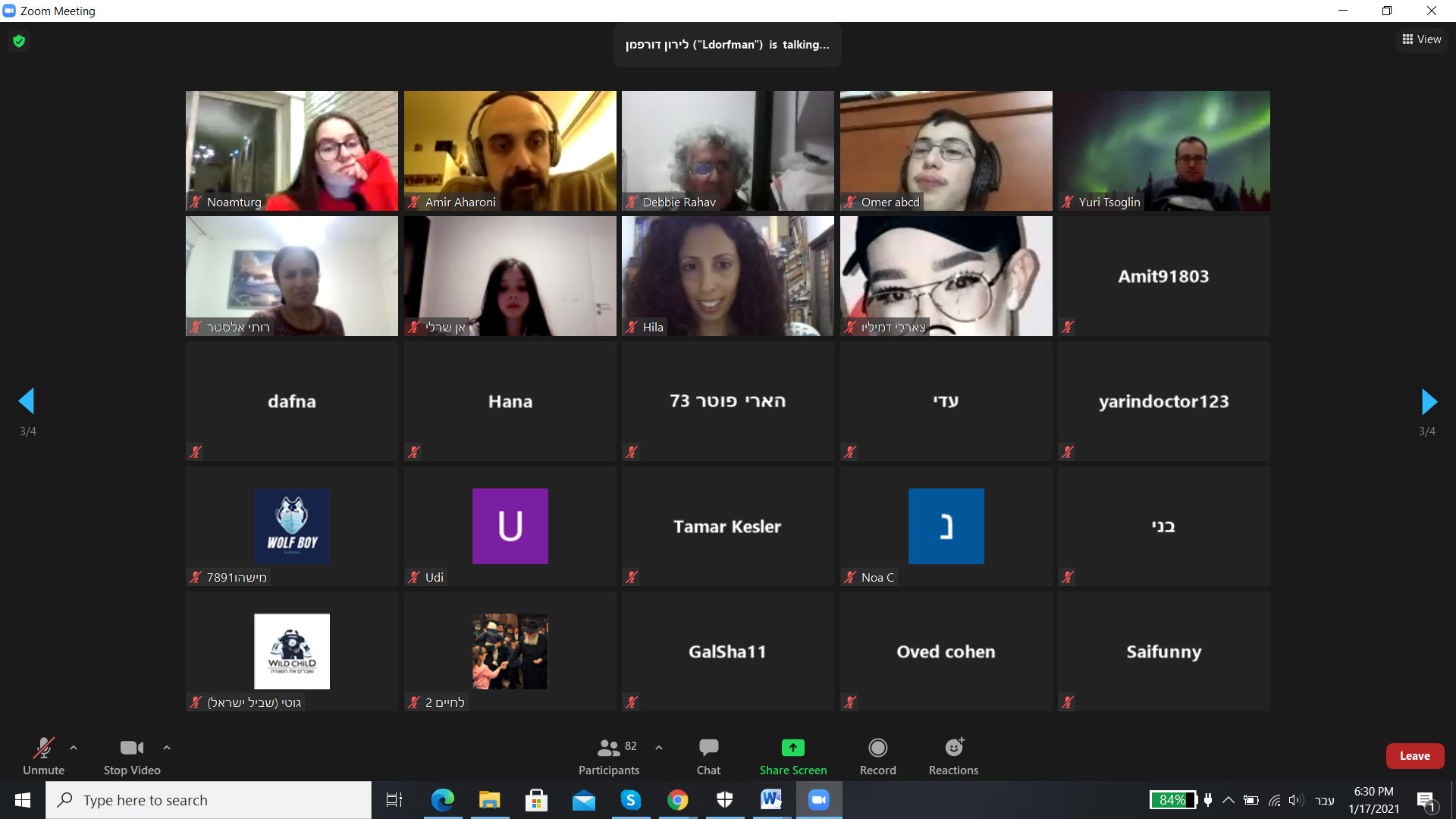
Opinion: Why is ending zoom meetings so awkward?
𝗪𝗵𝘆 𝗶𝘀 𝗲𝗻𝗱𝗶𝗻𝗴 𝘇𝗼𝗼𝗺 𝗺𝗲𝗲𝘁𝗶𝗻𝗴𝘀 𝙨𝙤 𝙖𝙬𝙠𝙬𝙖𝙧𝙙 ? First, you say bye and, during what seems like an eternity, have to gracelessly stare at the host and any remaining attendees as you all fumble around clicking the end meeting button or keyboard shortcut!
I realize there are more pressing issues to solve with #machinelearning but can't Zoom come up with a gesture or voice-activated feature to stop the meeting as soon as it's over to spare introverts like me from those clumsy moments. Does this happen to you? If so, what do you think should prompt the ending?
- 👋🏼 A wave gesture?
- 💬 The words "zoom end"?
- ⬅️ A slide left gesture?
- 🤷🏽 None: Suck it up!
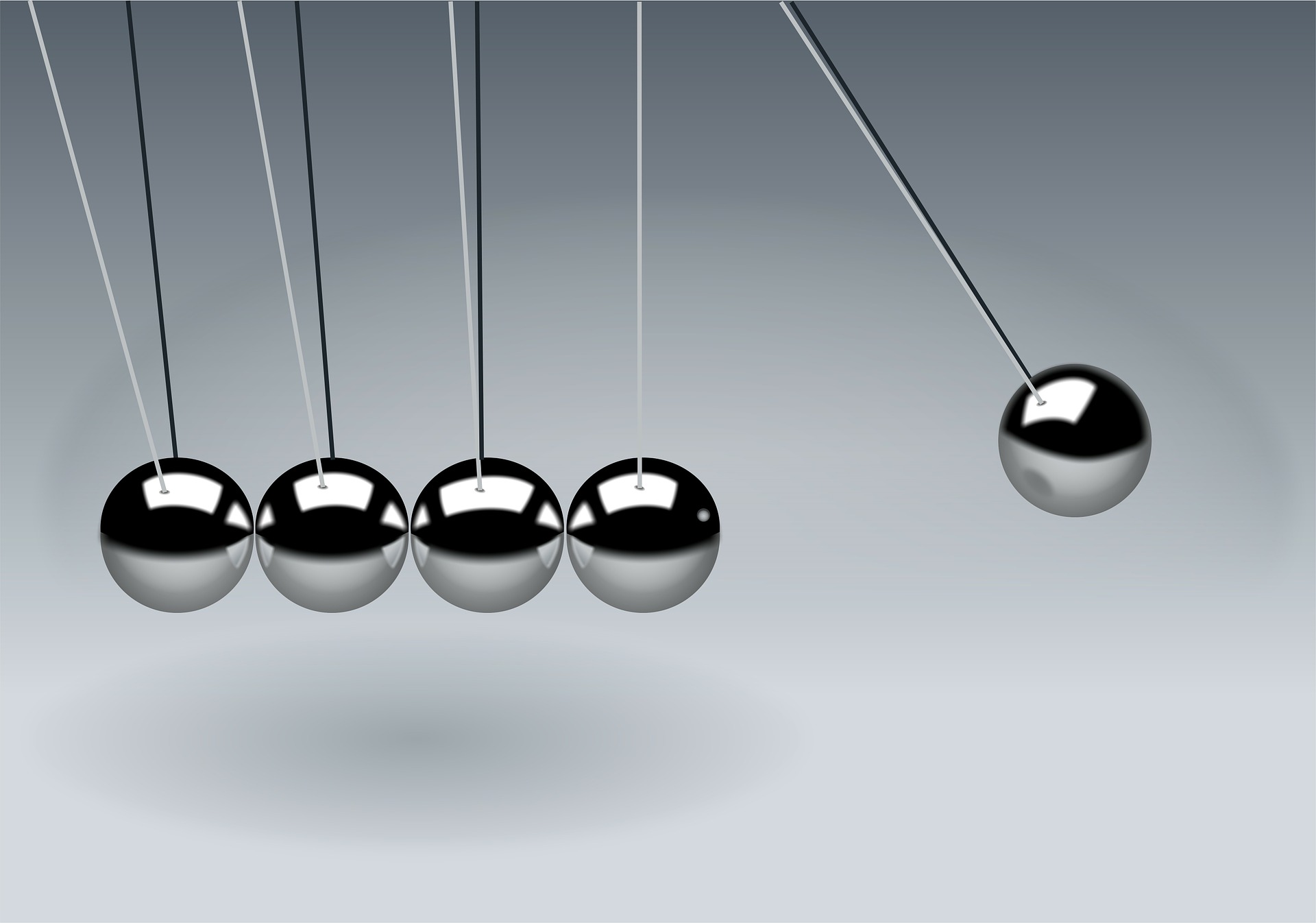
Causal Explanations of ML Models through Counterfactuals
We often hear "𝙘𝙤𝙧𝙧𝙚𝙡𝙖𝙩𝙞𝙤𝙣 𝙙𝙤𝙚𝙨 𝙣𝙤𝙩 𝙞𝙢𝙥𝙡𝙮 𝙘𝙖𝙪𝙨𝙖𝙩𝙞𝙤𝙣". And when working with data, it's easy to fall into this trap! Even aided by domain knowledge and complex models, it's often tough to disentangle both.
📈 I'm an advocate of 𝐈𝐧𝐭𝐞𝐫𝐩𝐫𝐞𝐭𝐚𝐛𝐥𝐞 𝐌𝐚𝐜𝐡𝐢𝐧𝐞 𝐋𝐞𝐚𝐫𝐧𝐢𝐧𝐠 (also known as XAI) because using approximations it can help understand models. However, I must accept that the most popular XAI methods rely on correlations, which is a significant limitation.
🔄 The solution to this problem is 𝐜𝐚𝐮𝐬𝐚𝐛𝐢𝐥𝐢𝐭𝐲 which yields a causal explanation rather than a correlation-based one. The authors of a recent paper (Leon Chou, Catarina Moreira, Peter Bruza, Chun Ouyang, and Joaquim Jorge), propose counterfactuals as a means to provide causability.
🤔 Counterfactuals are a good fit because they ask the question, "𝙬𝙝𝙖𝙩 𝙞𝙛?" which comes naturally to us humans and, given some properties, serves as a satisfactory causal explanation. There's a family of counterfactual methods that meet many of the properties. But, unfortunately, in recent years they have been overshadowed by other XAI methods.
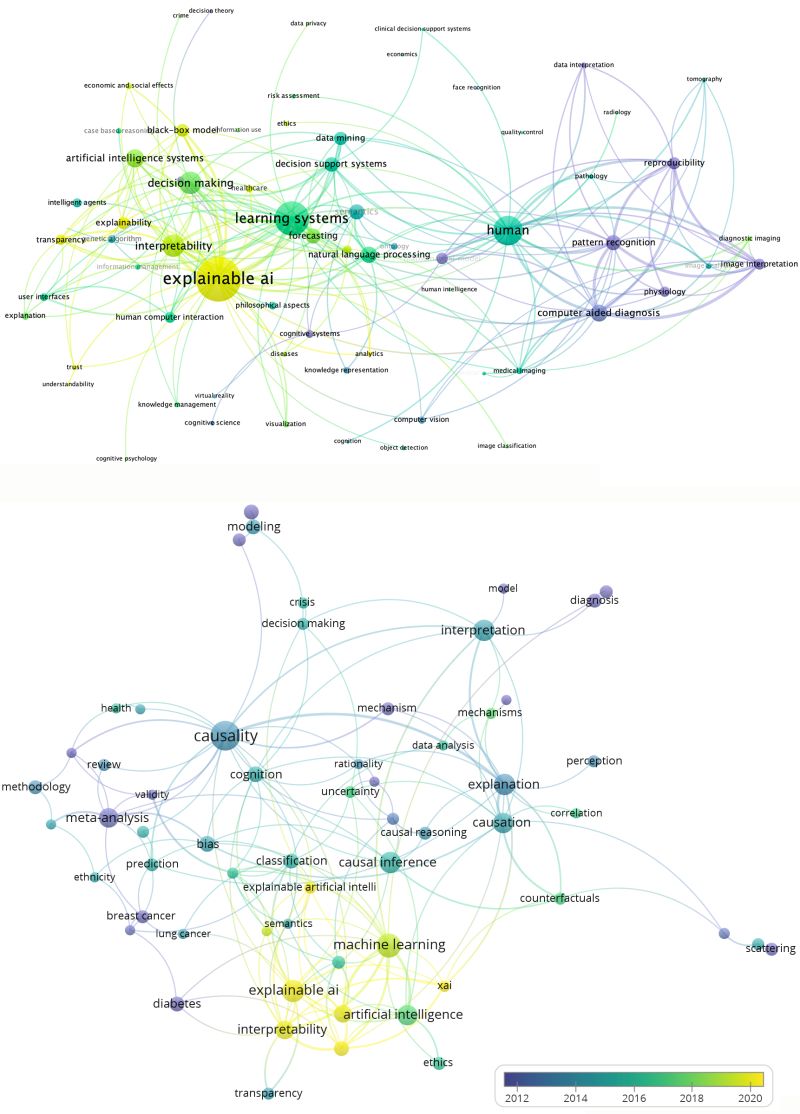
The authors of the paper performed a topic modeling and word co-occurrence analysis on academic research since 2012. It shows nodes for each keyword where size denotes frequency and color the most popular year. While it's good news that discussion has evolved from machine-centric topics such as pattern recognition to more human-centric such as XAI (see 1st figure), there are clear research gaps between causality and XAI - not to mention counterfactuals and causality (see 2nd figure). Check out their amazing paper for more details. Featured image by: Michal Jarmoluk from Pixabay
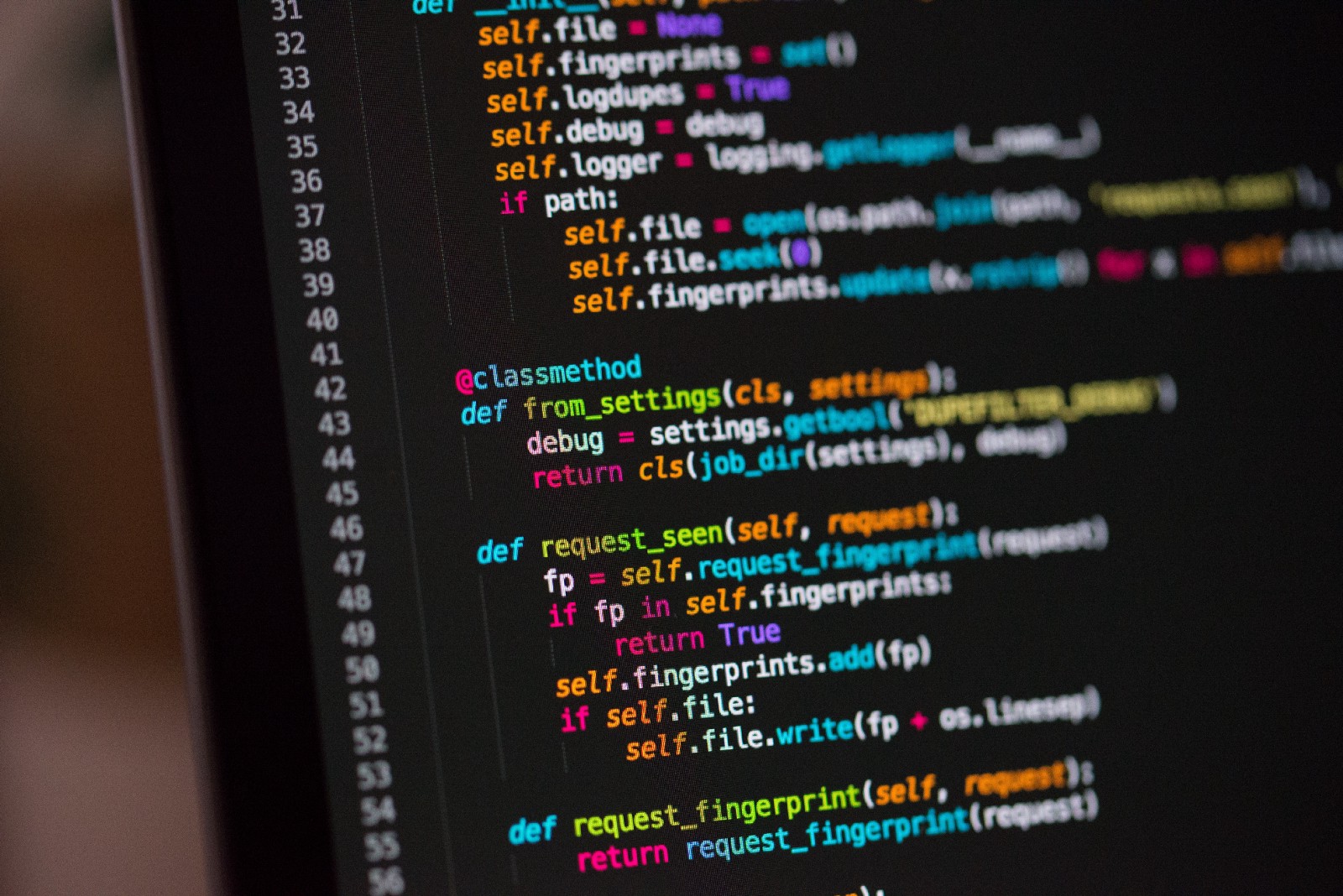
Python = Toolbox
#Python is merely a toolbox 🧰
It's a magical bottomless toolbox but a toolbox nonetheless.
Featured image by: 1840151sudarshan
Don't get me wrong. Tools are essential, but no tool should define the data science discipline. Tools come and go, but the fundamentals of our discipline don't.
For instance, 𝗰𝗮𝗿𝗽𝗲𝗻𝘁𝗿𝘆 didn't always involve power tools like circular saws, but it ALWAYS has involved 𝘄𝗼𝗼𝗱. So carpenters must first and foremost understand wood. It's many properties such as varieties, strengths, malleability, moisture, and grain. It's limitations and applications. Not to mention the language, diagrams and math used to discuss wood.
Likewise, the skill every 𝗱𝗮𝘁𝗮 𝘀𝗰𝗶𝗲𝗻𝘁𝗶𝘀𝘁 should have is understanding 𝗱𝗮𝘁𝗮. It's properties, limitations, and applications. Also how to effectively communicate findings to all audiences.
Articles tend to confound data science tools with skills, and data science books are mostly tool-centric, not 𝗱𝗮𝘁𝗮-𝗰𝗲𝗻𝘁𝗿𝗶𝗰 nor 𝗺𝗶𝘀𝘀𝗶𝗼𝗻-𝗰𝗲𝗻𝘁𝗿𝗶𝗰. And it's no wonder why I get messages from aspiring data scientists asking me what machine learning library they should learn first — tantamount to a novice carpenter playing with a circular saw on their first day! 🤷🏻♂️
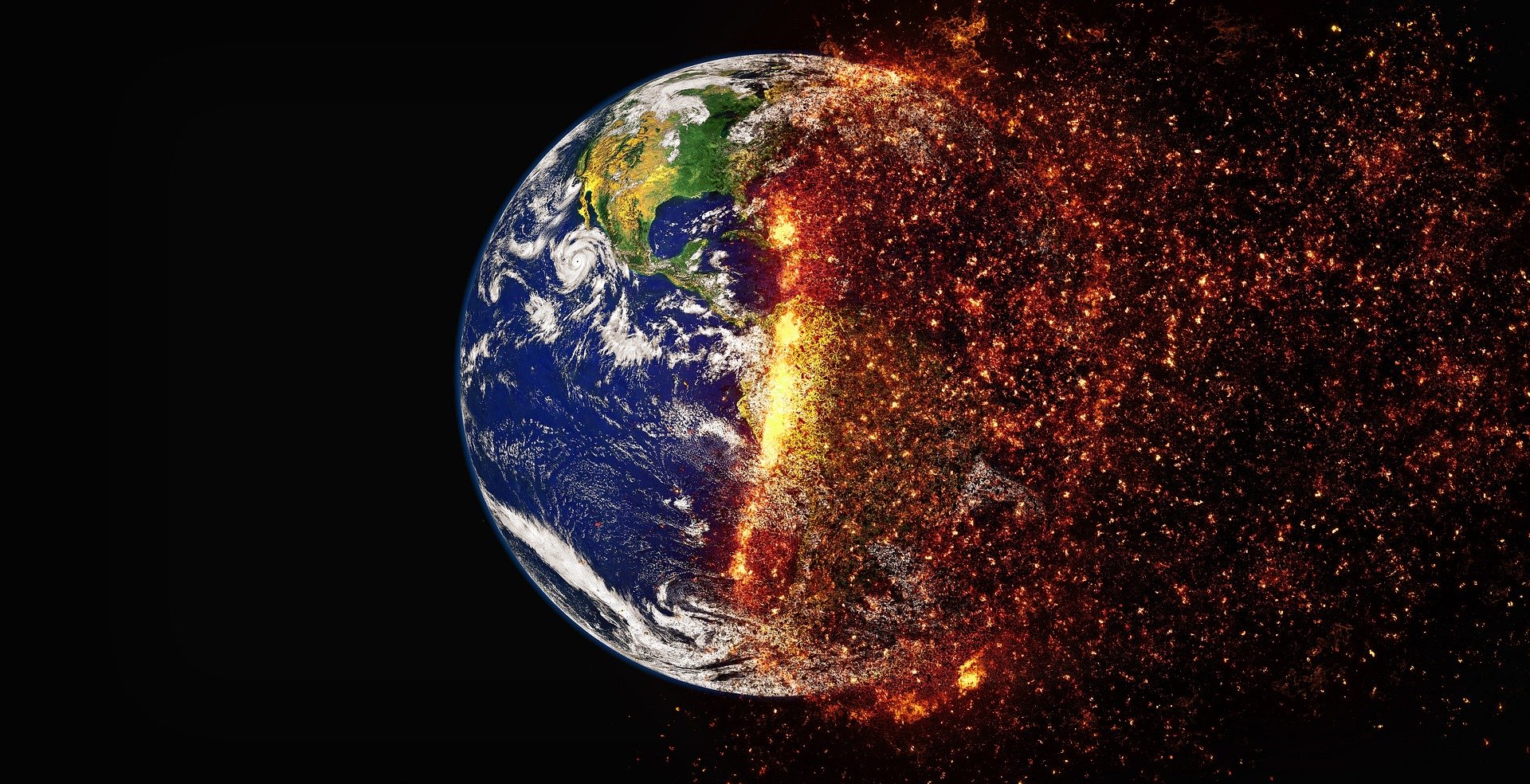
Review: How to Avoid a Climate Disaster
I recently finished Bill Gates's book on #ClimateChange. It's an urgent topic. And as a data scientist, any book that begins with a KPI of sorts and then spends the rest of the book breaking it down and explaining how to address each part will have me hooked! I applaud how he approaches some challenges. For instance, advocating for nuclear to address growing energy needs.
Above picture by: Alfred A. Knopf, Featured image by: Pete Linforth from Pixabay
That being said, it has some disappointing blind spots:
- 💸 𝗜𝗻𝗰𝗲𝗻𝘁𝗶𝘃𝗲𝘀: expects people to be only swayed by lower costs ("green premiums") — if only people were that rational!
- 🐟 𝗢𝗰𝗲𝗮𝗻𝘀: doesn't mention how large-scale fishing operations, not to mention container ships, are destroying the oceans, which sequester massive amounts of greenhouse gases (see 𝘚𝘦𝘢𝘴𝘱𝘪𝘳𝘢𝘤𝘺 on Netflix). He does mention mangroves but underestimates their role.
- 🌳 𝗘𝗻𝘃𝗶𝗿𝗼𝗻𝗺𝗲𝗻𝘁𝗮𝗹 𝗱𝗲𝗴𝗿𝗮𝗱𝗮𝘁𝗶𝗼𝗻: single-KPI approach cannot address how other finite resources already constrained and contaminated are magnified by climate change — while simultaneously contributing to it!
- 🗳️ 𝗣𝗼𝗹𝗶𝘁𝗶𝗰𝘀: no mention of how lobbying has slowed down progress on the climate change front and will continue to do so — unless stopped!
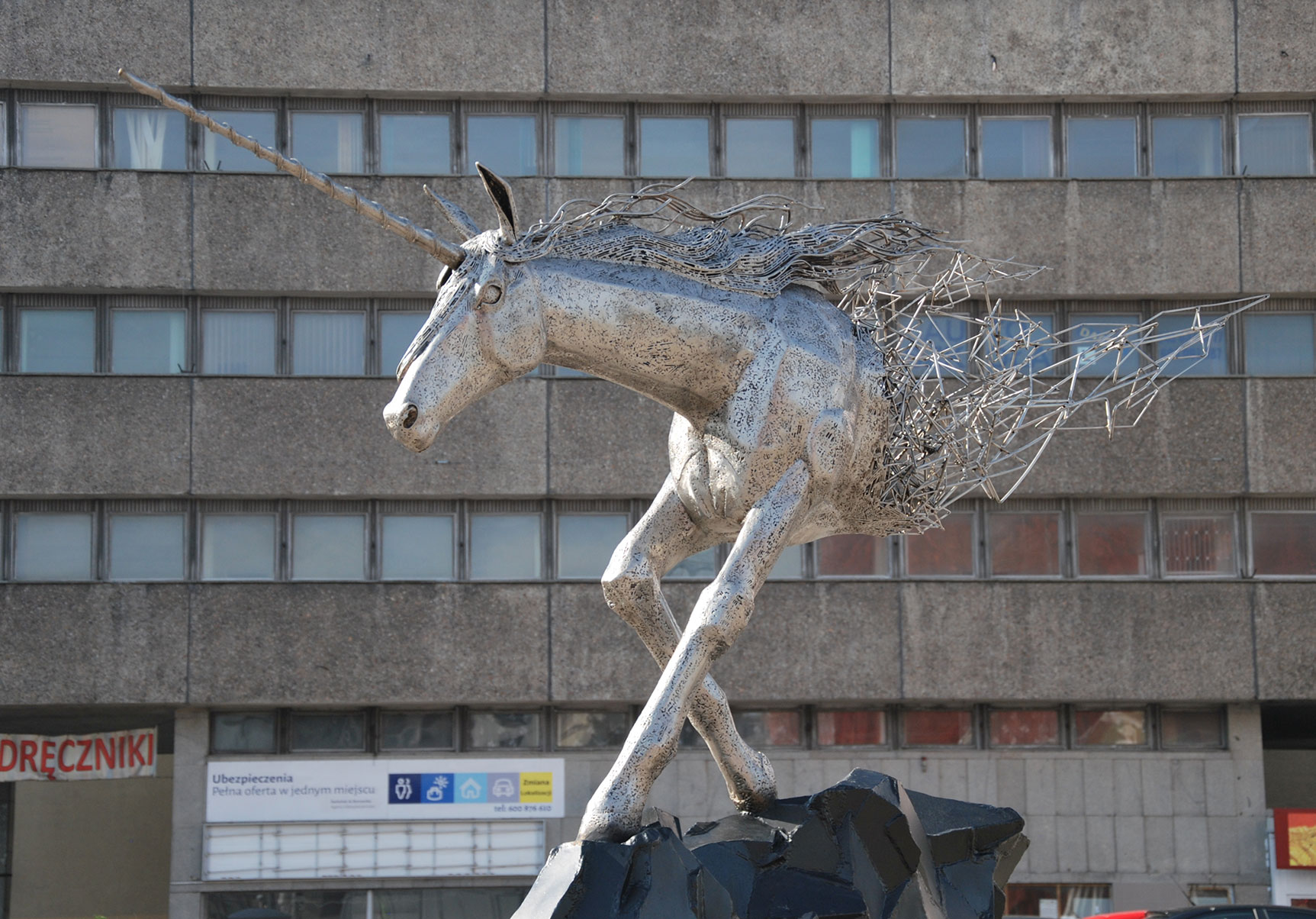
Confession: I Hate Unicorns
Today is national 𝐔𝐍𝐈𝐂𝐎𝐑𝐍🦄 day. Yes, there REALLY is such a thing!
And I HATE unicorns! Not the mythological creature, just the label used to describe some data scientists and startups. It's so darn pretentious.
In ancient descriptions by Greek historian Ctesias (400 BC), the creature is associated with rareness, purity, beauty, protection, and salvation, and even today, it still carries these connotations. That label suggests a level of magnificence beyond reproach — and collaboration. As a data scientist who once co-founded a startup, I find that data science and entrepreneurship are among the worst domains to apply that label. I believe in the suggestive power of words, and if we are to challenge ourselves, unicorn status should remain unattainable.
Featured image by: Tomohiro Inaba
In my experience, data and startup endeavors require ample collaboration, humility, and constant challenges. They involve hiking steep mountains with no summit, and you are not alone because, out in the real world, they are inherently team sports. They also operate outside of your comfort zone, and if you get too comfortable, watch out! Any time now, you will tumble down the mountain!
What do you think? Do you think it is an appropriate label?
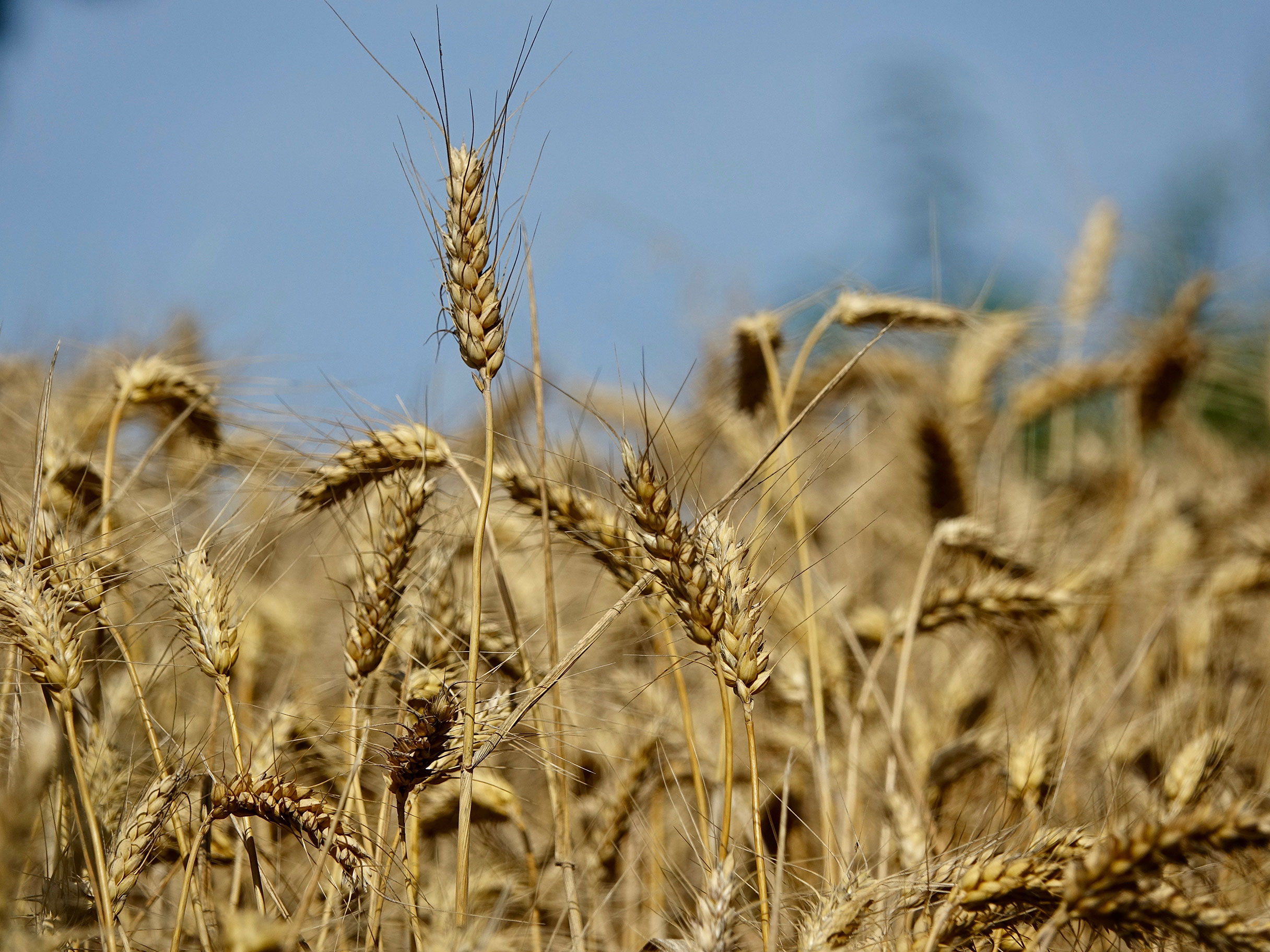
The Long History of Data in Agriculture
Today is National Agriculture Day, and I'm a data scientist in 𝗮𝗴𝗿𝗶𝗰𝘂𝗹𝘁𝘂𝗿𝗲!
When fellow and aspiring data scientists approach me they seem surprised that something as "modern" as data science and as old as agriculture to be uttered together!
But it's only natural for data and agriculture to be paired together. Agriculture is about 10,000 years old — the world's oldest (and largest) industry. However, it was very small-scale for thousands of years. Modern agriculture was born in southern Mesopotamia, present-day Iraq, where the Sumerians supercharged it by inventing NOT ONLY the wheel and the plow but ALSO data!
Above picture by: MET Museum, Featured image by: Del Adams
Indeed, the Sumerians invented the first accounting and writing systems to keep records for inventories of grain harvests, storage, and transactions. This cuneiform tablet is around 5,000 years old and is one of the oldest known written artifacts. It concerns the distribution of barley and emmer.
Ancient scribes weren't just bookkeepers and accountants. Their data would help answer questions like when to plow the fields? How much will the crops yield? And what fields are most productive? In fact, the first farmer's almanac is Sumerian.
Is it a stretch to say they were the first data analysts? Do you agree?