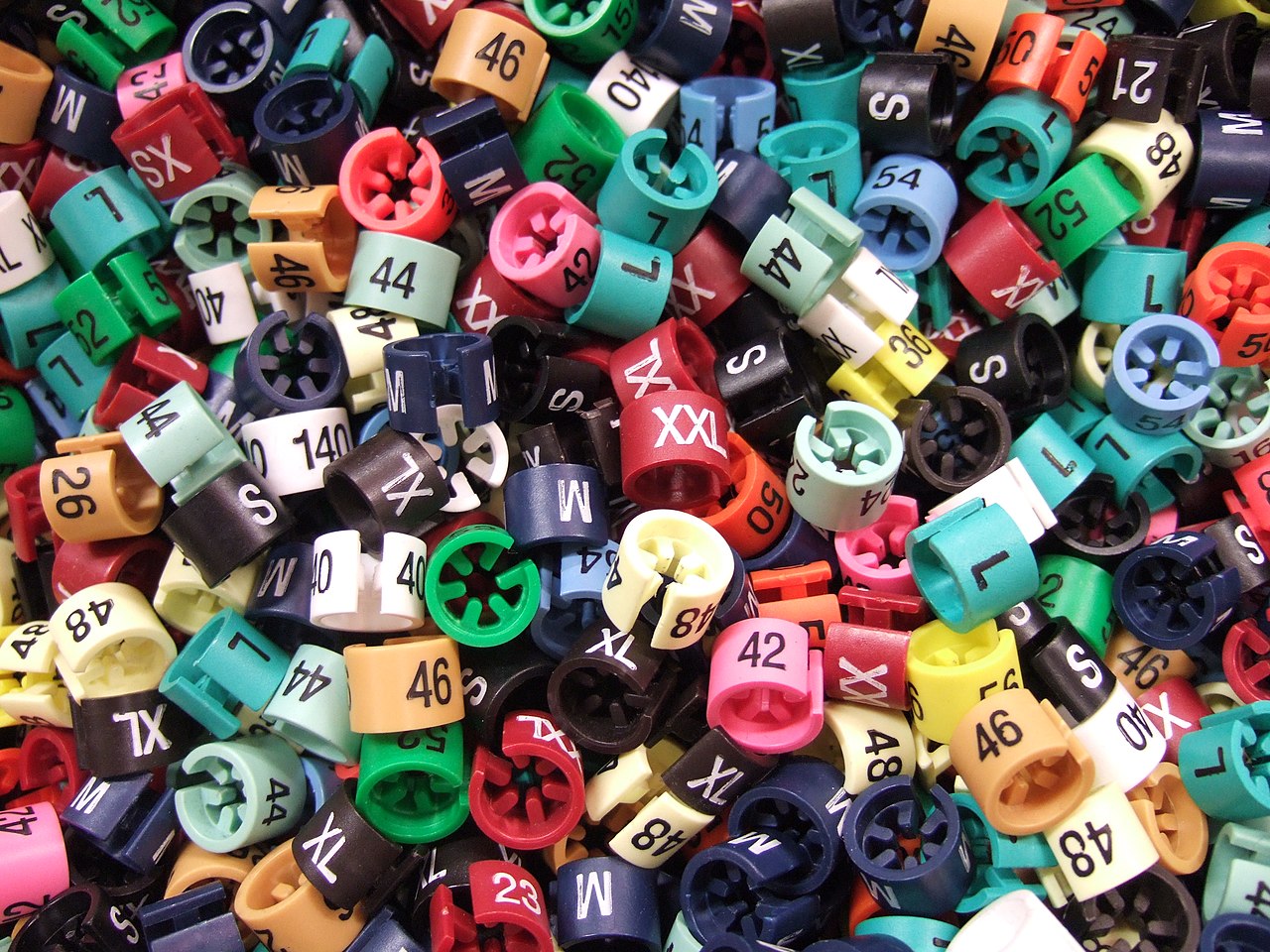
The One-model-fits-all fallacy
A common mistake in Machine Learning is having a "go-to" model because it usually fits the data well, which is a big assumption because it might have an entirely different distribution. It's like expecting someone of more or less the right size, say medium, to fit on all the clothes in your closet!
On the other hand, a data-centric view calls for finding a suitable model for the variations of data you can reasonably expect in a collection. It's like finding the person that can wear all the clothing in your closet. I guess that would be you 😅
Just as there's no one size fits all in clothing, with machine learning, no model fits all.
Above picture by: Serg Masís, Featured image by: Ralph Aichinger @ Wikimedia
I'm just having fun with the clothing analogy, but it always doesn't work since clothing-fitting is primarily about the person. On the other hand, the data-fitting process is more about the data than the model. Therefore, the focus should always be on the data first and foremost — data quality and consistency. Then, find the right model. After that, iterate the data, not the model.
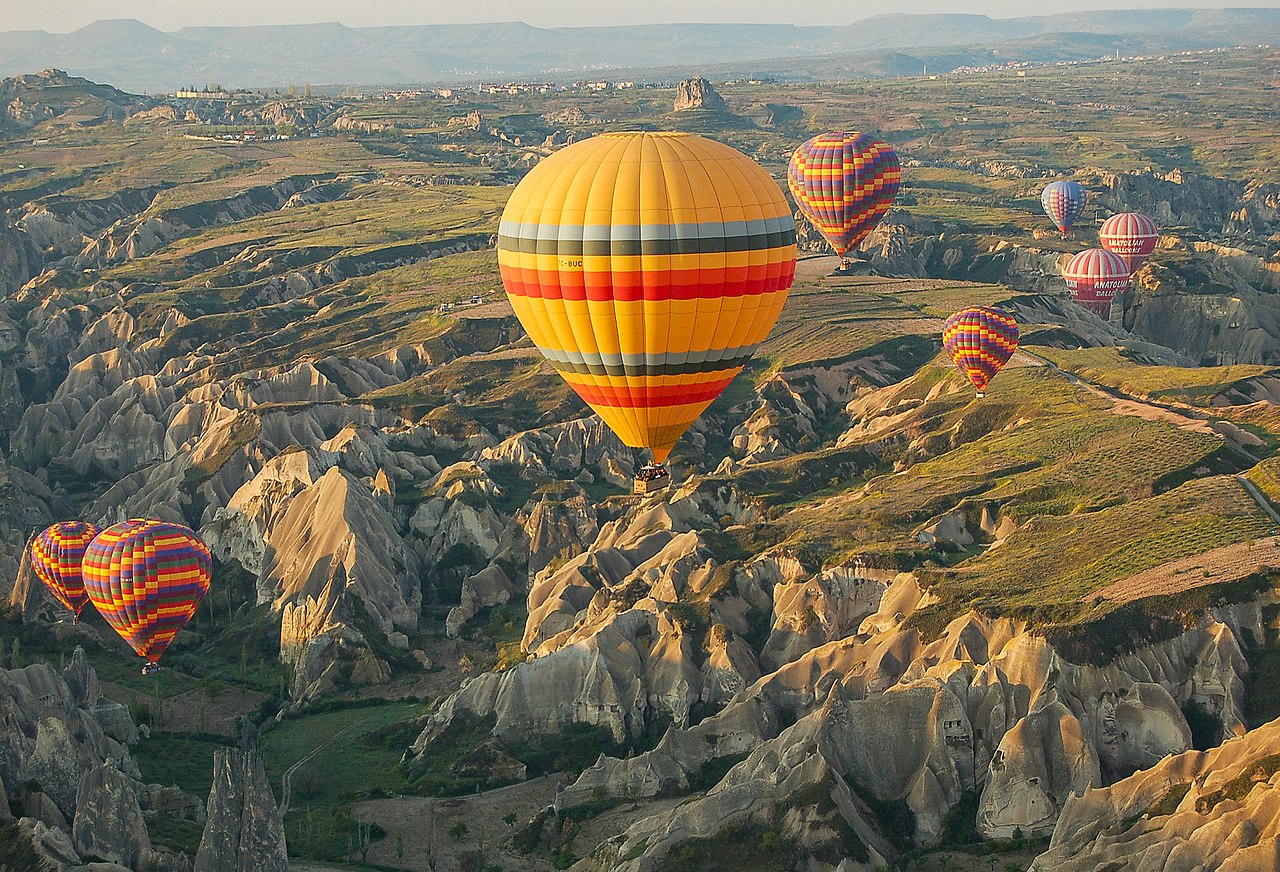
How to “make it” in any field?
Last week at the Open Data Science Conference, a young volunteer graduate student asked me what she needed to do to "make it" in Data Science. I told her to keep doing what she was doing. Conferences are a great way to learn new skills and meet new people. And nothing beats in-person events for networking. So many of the colleagues I can now call my friends I met at an event.
Then, she asked me in what other ways conferences have helped me. I said, "I was once a graduate international student volunteer at ODSC just like you, and I don't think I'd be speaking here today had I not learned other skills that are not taught in courses — at conferences!". She was in disbelief and asked me what skills?
I told her that, unfortunately, most students obsess over academics, such as maximizing GPAs and publications, but they genuinely miss out on understanding the following:
💬 How do practitioners talk about data? It's a different language than that found in academia. For instance, it focuses on scalable deliverables and proving their consistency and performance with quality control. Speaking in these terms at job interviews will give them an edge over other candidates.
🔥 What methods/topics are popular in industry right now? In an ever-evolving field, it's essential to know what to focus on so you can tailor your portfolio and resume to match what employers are looking for.
🎯 What inspires you? It would be best if you had examples of how data science is leveraged in many fields to decide where to focus your efforts. Maybe you don't like what you thought you liked. Perhaps you found a niche you want to explore further.
🎨 How to think creatively about solving problems with data? There are countless ways to solve the very same problem correctly with data. Don't let anybody tell you that there's one optimal way. But it would help if you saw how other data scientists approach problems to spark creativity.
The volunteer nodded in agreement. She had been learning these things without knowing that she had! And it truly hadn't dawned on me that what seems like such a long way from data science conference volunteer to author and speaker was made shorter by eagerly attending every data science event I could. Learning is not always about toiling in the coursework but attending social events such as conferences, meetups, and hackathons.
Above picture by: Serg Masís, Featured image by: Ratta Pak @ Wikimedia
So, to sum up my advice to any newcomer: you need more than textbook knowledge to make it in any field, so you have to have the proactiveness and tenacity to seek opportunities to put yourself out there among your peers (much like the volunteer did). And that will rise you up!
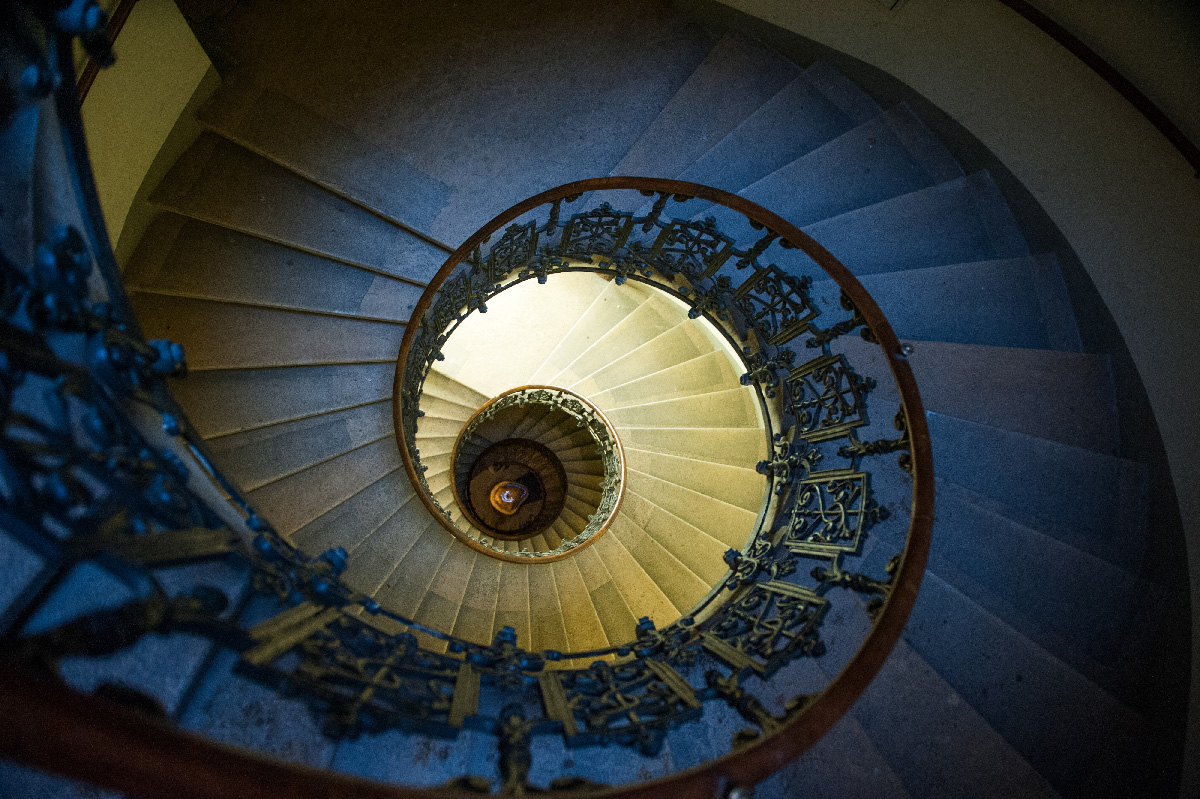
The Downward Spiral of Bias in Machine Learning
What motivated me to become a data scientist was to help improve decision-making with data. Machine learning models are one of the many tools available to do so. A naive description for supervised learning is that it takes data that represents reality and, with it, trains a model that makes predictions. In turn, if these predictions are deemed good enough, they can help improve decision-making. Simple! Right?
However, this process has several fatal assumptions:
⚖️ You can trust the data generation process. If it's biased, then the data can't be trusted either. And biased data can only make a biased model.
🤖 The choices you make as an ML practitioner, do not impact model bias. In fact, every choice you make, from feature selection to model selection and tuning, can potentially amplify bias or create bias where there was none.
📈 If the predictive performance is high, then the predictions can be trusted. Nope. What if the model always misclassifies in specific cases that disproportionally impact a group? What if the training data doesn't match the distribution of real-world conditions? What if the ground truth is biased? What if even when the predictions are good, they are misunderstood or misutilized by users in such a way they become biased decisions?
🔮 You want to predict based on the "reality". Don't you want to improve decision-making? If the past is biased, then why not predict for a better future!
Above picture by: Serg Masís, Featured image by: Mstyslav Chernov @ Wikimedia
When left to its own devices, each model can become a bias amplification machine. As a result, and in aggregate, AI systems can replicate human biases at an unprecedented scale. Fortunately, there are methods to mitigate model bias in all steps ML practitioners are involved: pre-processing methods for the data, in-processing for the model, and post-processing for the predictions.
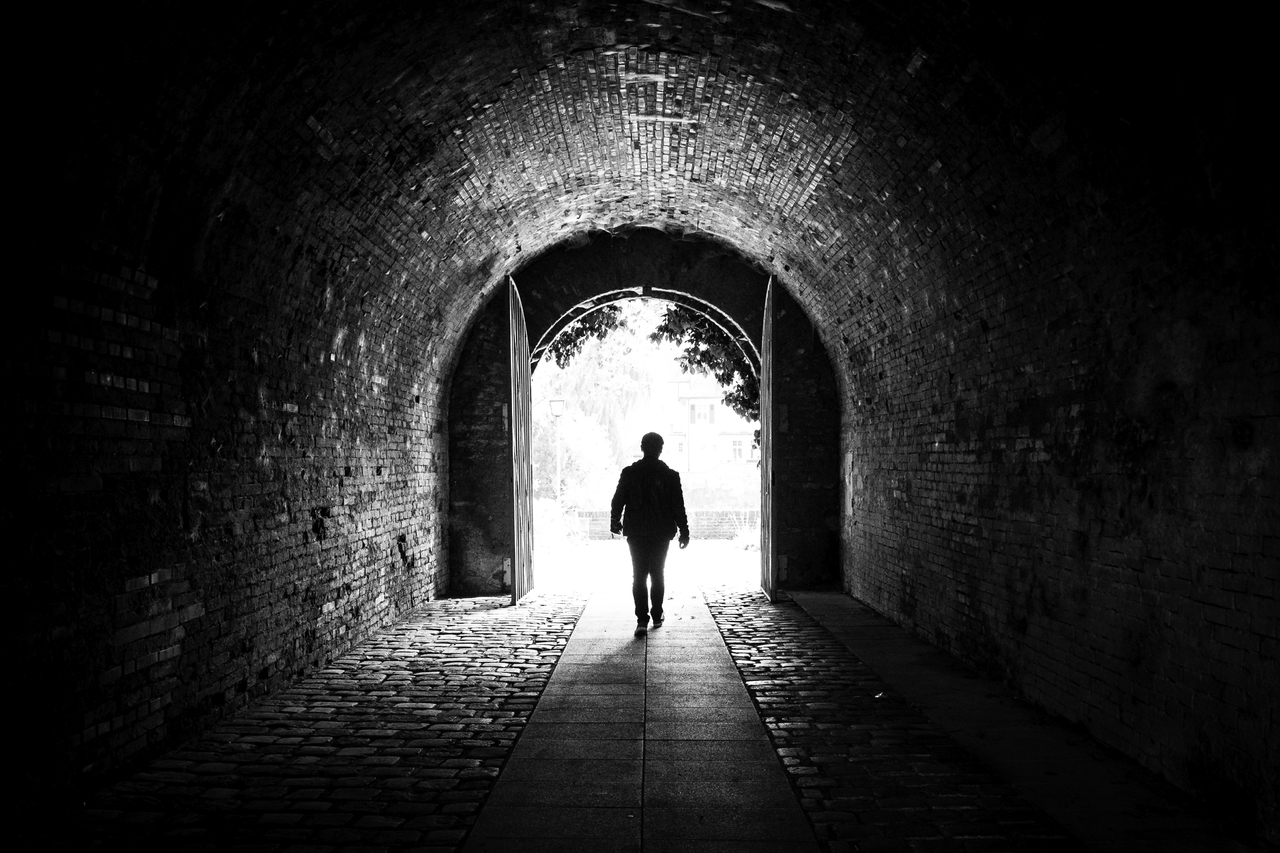
You are not alone!
Imposter Syndrome or imposterism happens when your perceived ability (confidence) is lower than your actual ability (competence). It causes you to feel like a fraud despite your best efforts to belong. 🙈 I've had this on and off throughout my career. And I used to think it was something to feel ashamed of, but I have realized it's much more common than you think.
📚 There's so much to learn in data science that it's easy to feel like a phony despite your best efforts to keep up. Talk shop with just about anyone in our field, and they'll bring up a method that you've never tried or a book or paper you've never read.
🤡 As bad as imposter syndrome can feel, over-confidence (like over-fitting) is much worse because it can lead to foolish mistakes. Unfortunately, newcomers tend to do this — a phenomenon called the Dunning-Kruger effect. In the worst cases, it will not subside till it's too late, as it did for Elizabeth Holmes, the infamous CEO of Theranos.
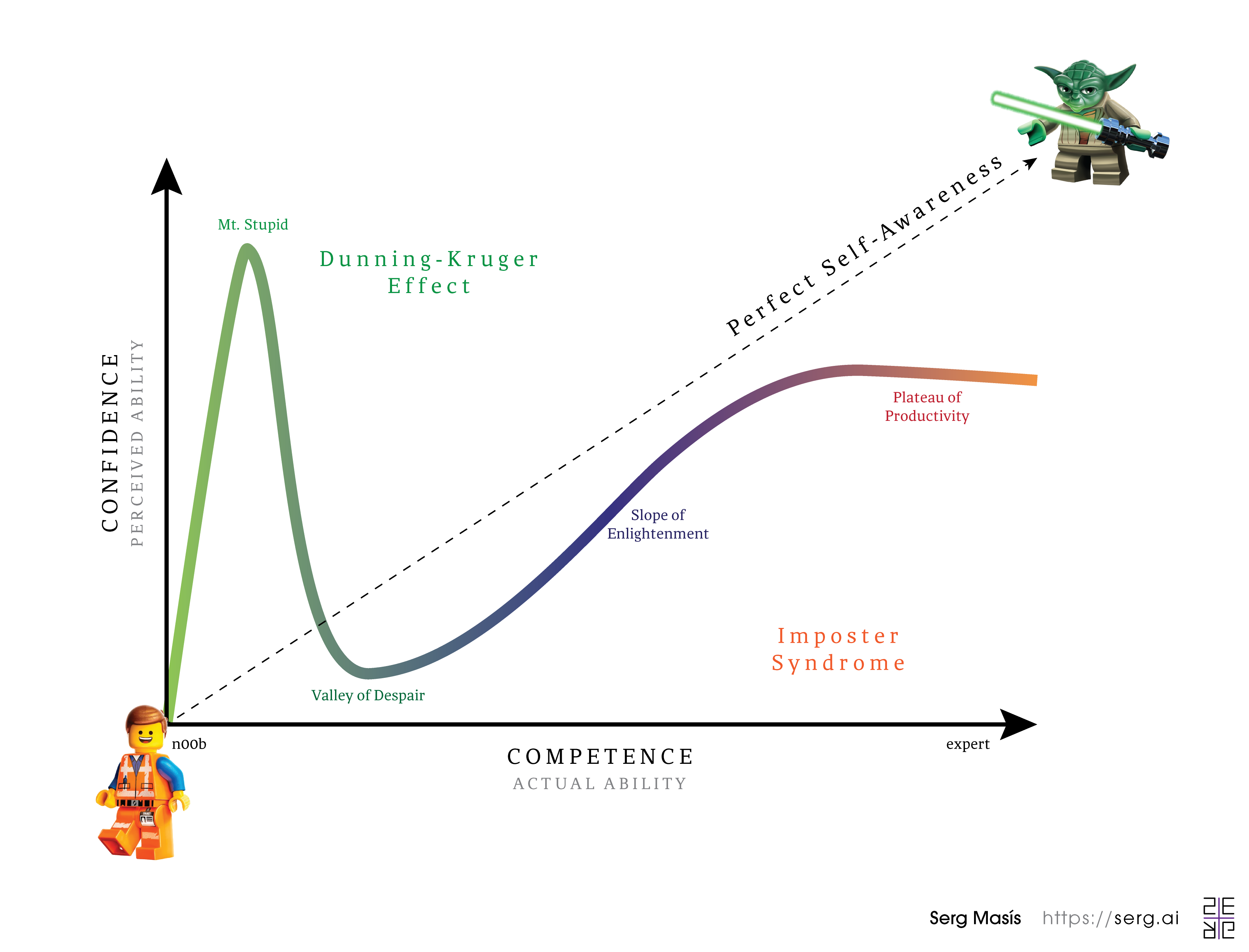
Above picture by: Serg Masís, Featured image by: PxHere
🎯 In any case, shortchanging yourself from some confidence is necessary because it fuels the humility and curiosity to continue learning in an ever-expanding field. However, I've understood that I shouldn't expect myself to know everything and let feelings of inadequacy paralyze me. Instead, I should avoid comparisons with others and channel any legitimate concerns into concrete goals.
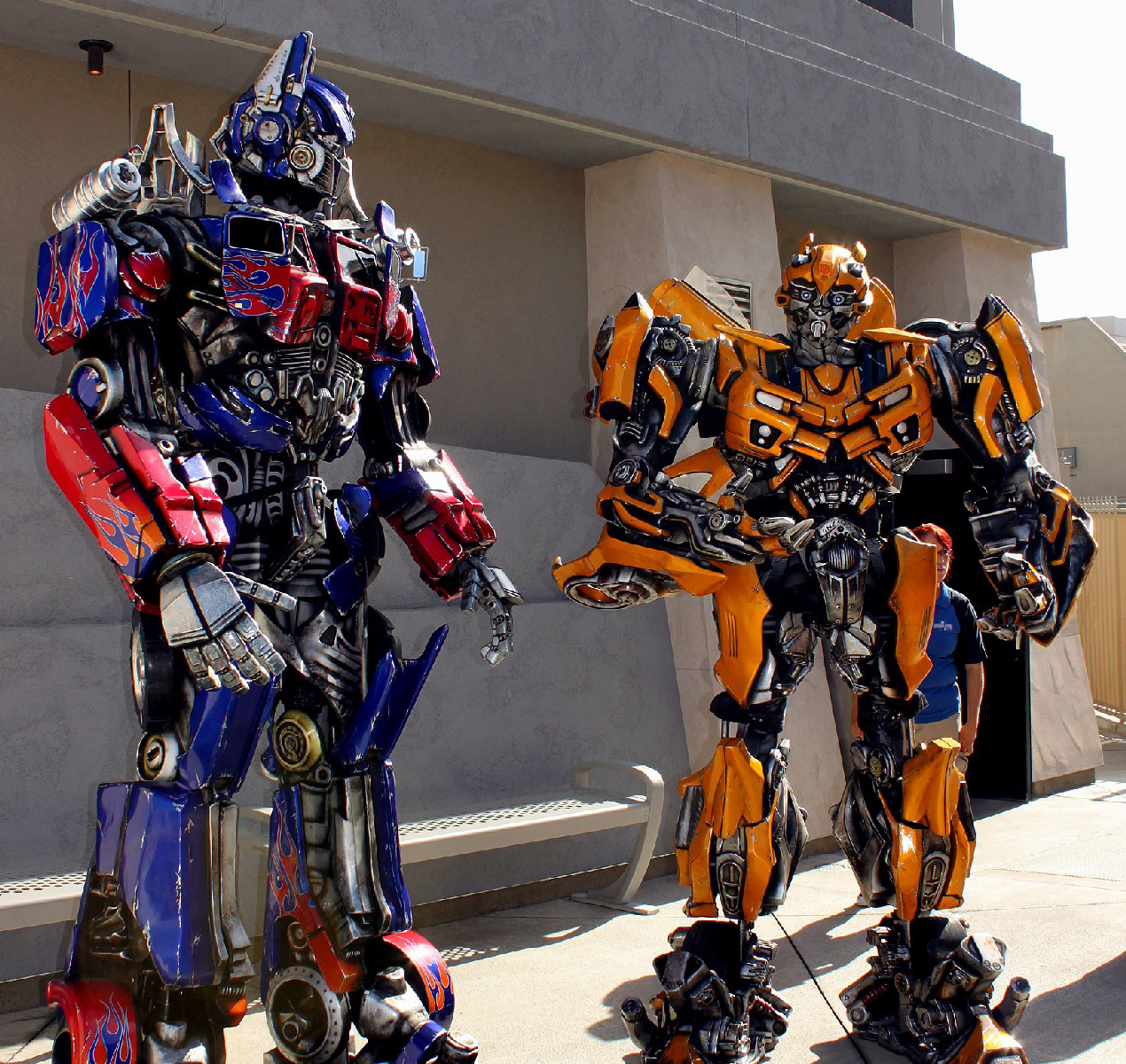
With Great Power Comes Great Responsibility
I'm working on a new chapter on interpreting NLP Transformers for the 2nd edition of my book. This topic was the most popular one in a poll I conducted on LinkedIn. But, to be honest, initially, I wasn't sure about doing it.
🔥 Transformers get a whole lot of attention (no pun intended). However, while solving the bottleneck problem recurring neural networks (RNN) have, they have unleashed an arms race to train ever-increasing larger models with even bigger corpora — and from the Internet. I'm not convinced this is the way to go because language is riddled with bias. So all the more reason to learn to interpret these models! Because only through interpretation can we understand the strengths and weaknesses of a model and develop strategies to solve them.
👍🏼 And with this thought, I convinced myself to include this chapter, and I'm very excited about how it's turning out! (and so is my furry four-legged assistant)
Above picture by: Serg Masís, Featured image by: prayitno @ Wikimedia
📖 Anyway, I can't believe it's been a year since the bestselling Interpretable Machine Learning with Python (1st edition) hit the shelves.
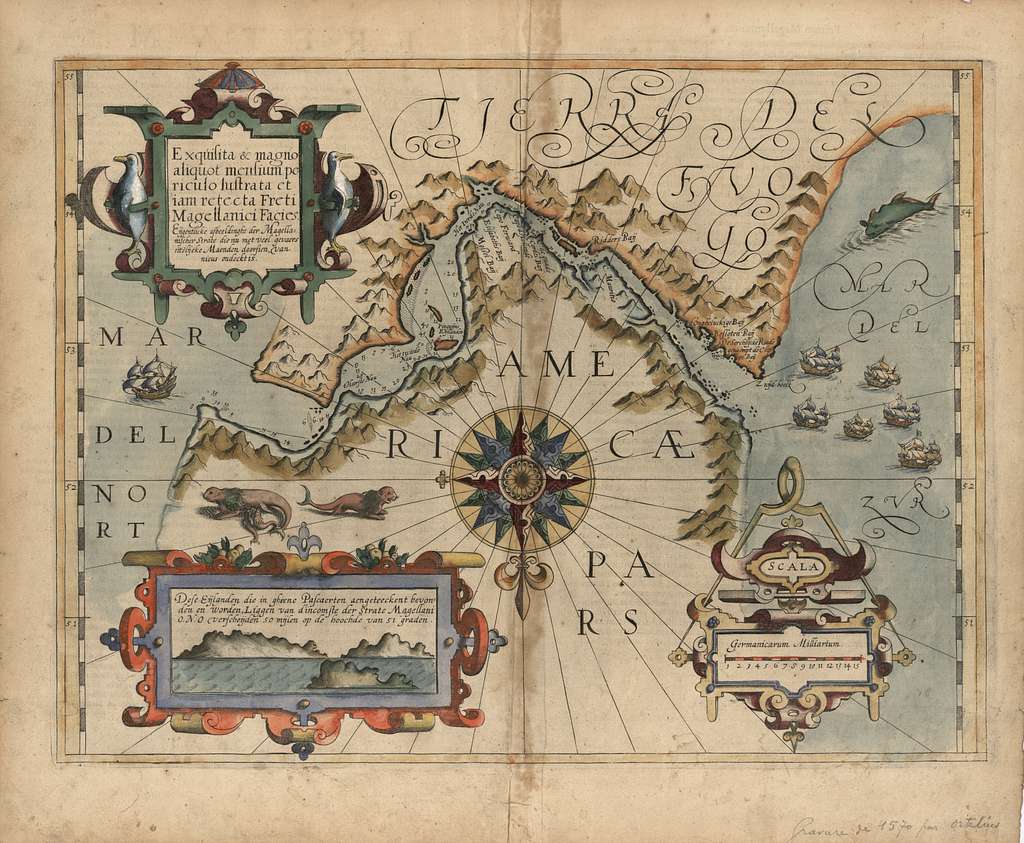
Navigating the World of Data Science
Data science is a vast body of knowledge encompassing science, business, and engineering, and there's still a lot yet to explore, like the oceans in the age of discovery. Recently in a few events for entry-level folks, I have shared my thoughts on navigating it with this old-fashioned map that I made.
First of all, I encourage people to practice sailing around Sea of Probability and Statistics, while also learning how to extract value connecting it to the business side. Only then, drift to the Bay of Computer Science, practicing around programming, data wrangling, and MLOps to name a few.
Then, I recommend that the uninitiated seafarer only ventures into deeper and stormier waters when they have mastered calmer and shallower ones. There be dragons and rough waters! In particular, the area around Deep Learning Point is dangerous for beginners, despite how accessible it seems.
Above picture by: Serg Masís, Featured image by: Gerardus Mercator (1611)
Lastly, other exciting seas such as Actuarial Science, Econometrics, Financial Quantitative Analysis, Biostatistics, Geographic Information Systems, and Operations Research are typically not seen as part of data science, but very skilled data sailors have learned the ropes in those waters. It's definitely worth exploring these areas should you care to work in finance, insurance, logistics, economics, biotech, agriculture, and many other industries or disciplines that connect to them.
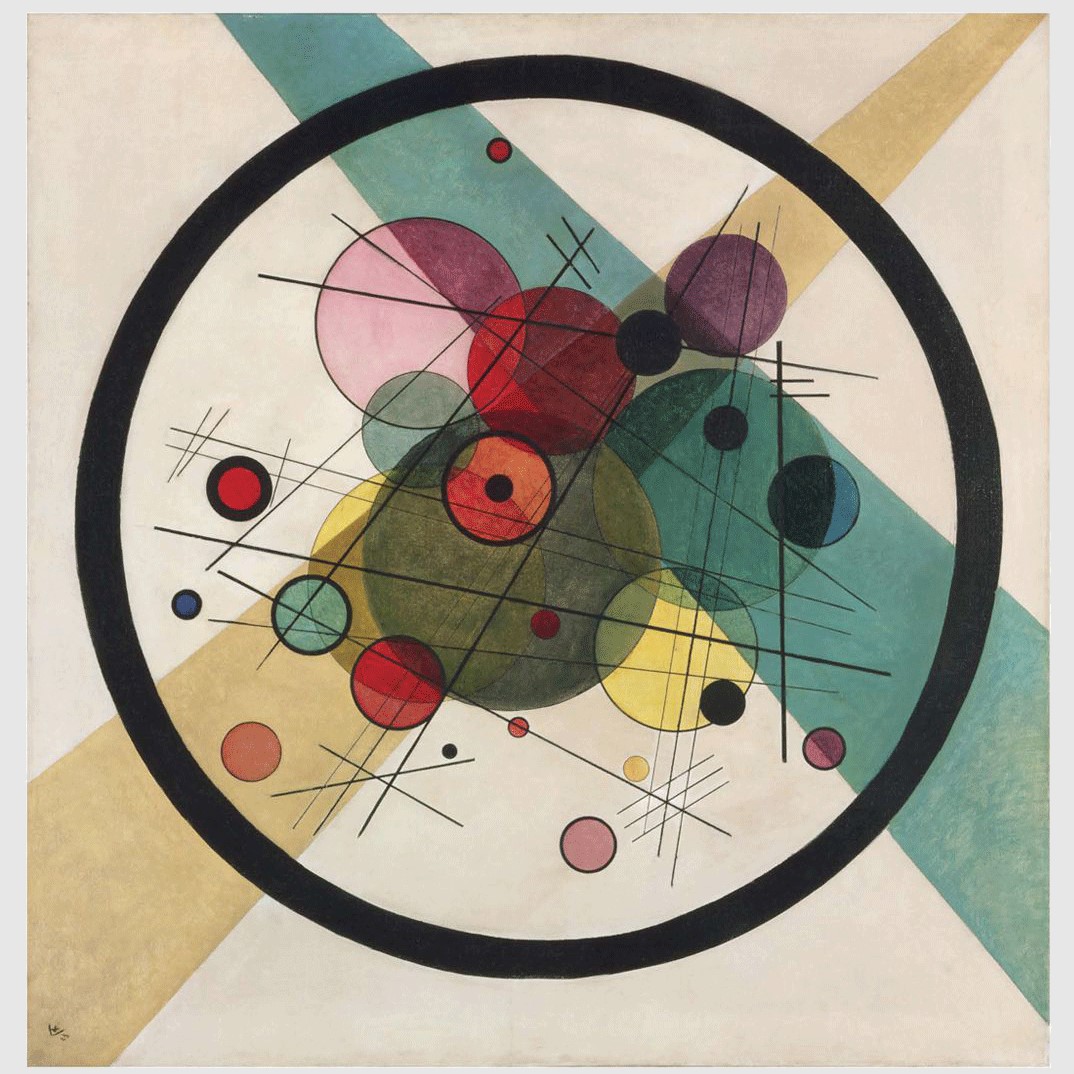
Happy π Day!
Here's a pie chart of how pie charts are called worldwide. Did you know they are called "Pita diagrams" in Greece, "Camembert diagrams" in France, 🍕 "Pizza graphs" in Portugal, and "Cake charts" in China and Sweden? In fact, a quarter of the world uses the word "cake" while another quarter uses words like "round" or "circular" to describe it. Such is the case in all the Spanish-speaking world.
Featured image by: Vassily Kandinsky (1923) titled "Circles in a Circle" retrieved from Wikimedia Commons
🥧 Yet, pie is still king, but I suspect it's only because the British Empire once held sway over 23% of the world's population. Then, in the 20th century, the United States has propagated English terms further, as some regions were starting to adopt statistics. Perhaps more imaginative and more localized terms would describe a pie chart otherwise.
Data visualization embodies cultural considerations and preferences, from colors to shapes to proportions. And I think this extends to the language used to communicate about it.
What do you think? Is there something to interpret? What is your favorite term?
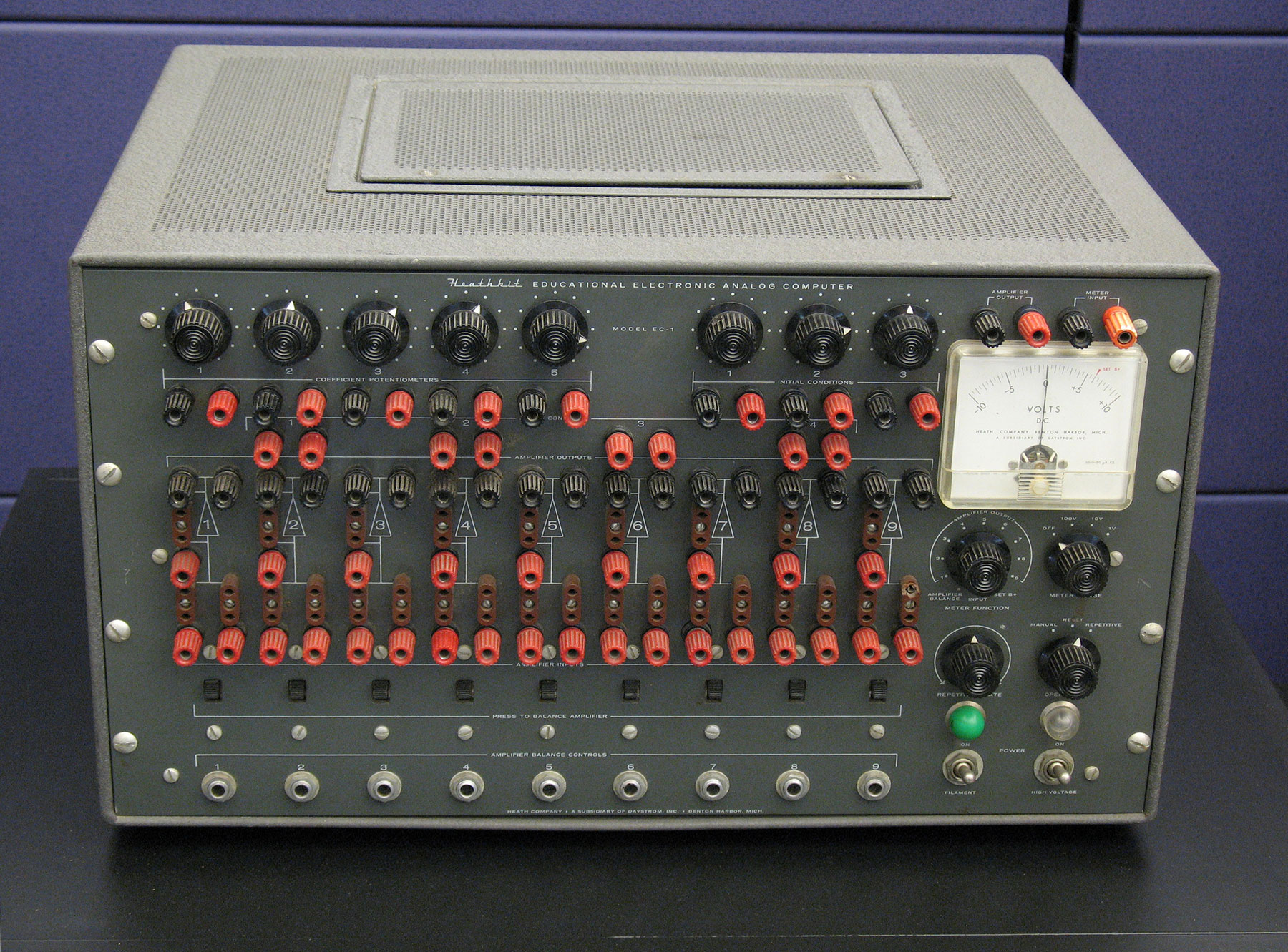
Analog computing makes a comeback
A startup, Mythic, has created analog chips that perform linear algebra for AI by solely leveraging the properties of voltage and conductance! This is exciting news because when Moore's law peaks in a couple of years, analog chips may be the scalable and efficient drop-in replacement for the digital chips we use today.
⚡ The attached clip is a small portion of a recent Veritasium video that explains this new technology. It shows how these chips can produce 25 trillion math operations per second (teraflops) with only 3 watts! Much more efficient when compared to the NVIDIA Titan V GPU, which can produce 110 teraflops at 250 watts.
Featured image by: The Heathkit EC-1 Educational Analog Computer (1960) retrieved from Wikimedia Commons
🤖 Efficiency matters since a massive carbon footprint is one of the most significant vulnerabilities and negative externalities for both artificial intelligence and blockchain. And it's not just SOTA technologies! Compare the wattage of any computer to what an adult human brain consumes: 12-20 watts.
🧠 Indeed, the brain is efficient because biological neural networks orchestrate both analog and digital signaling seamlessly. Moreover, I'm convinced that biological systems and processes inspire the best human engineering. Biomimicry makes for the most robust materials, efficient design, structural integrity, and much more. So why second guess 3.7 billion years of evolution?!
🔮 As promising as analog computing seems, it's hard to tell what will happen in the next decade. Neuromorphic computing will go beyond just changing the signaling of the hardware but also attempt to mimic biology through different use of materials and architectures. We also have quantum computing which might just blow everything out of the water but may never be consumer-facing.
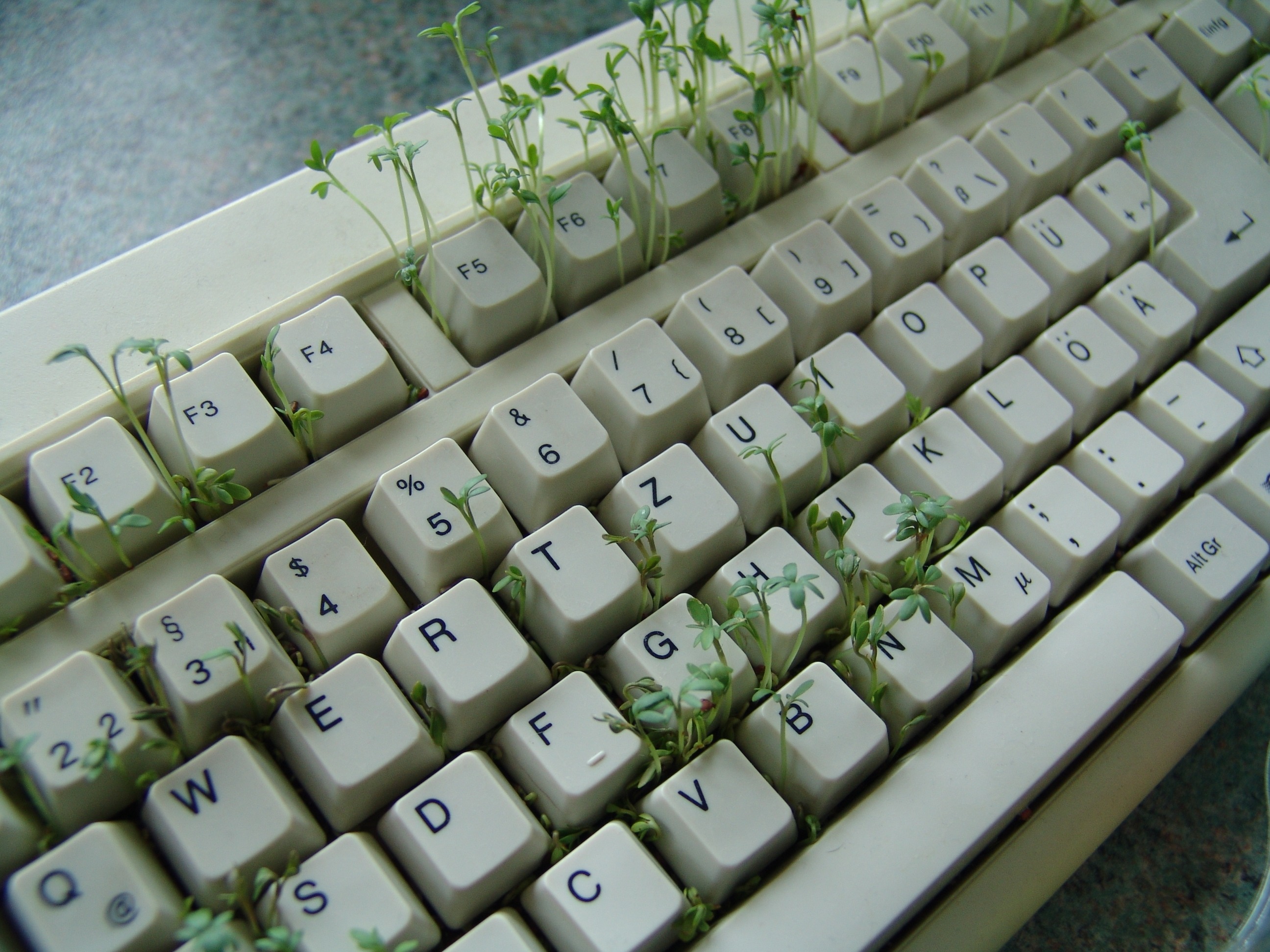
Rate my setup…
To me, the best thing about my setup, hands down, is my puppy 🐶 . Whether on my lap or roaming around on the floor of my office, she keeps me company while I work. And right now, she's helping me with a deep learning project.
Featured image by: PxHere
I can also feel the difference she makes. I feel more optimistic and productive 😅 . Pets have been proven to reduce stress and anxiety, boost self-esteem and empathy, and mitigate depression. Some immediate effects of petting a friendly dog include lowering blood pressure, slowing heart rate, relaxing muscle tension, increased oxytocin, and more regular breathing. Mental health matters!
For instance, now that I'm working on the second edition of my book "Interpretable Machine Learning with Python" 📖 and facing crippling writer's block, putting her on my lap and petting her for a while helps me overcome anxiety.
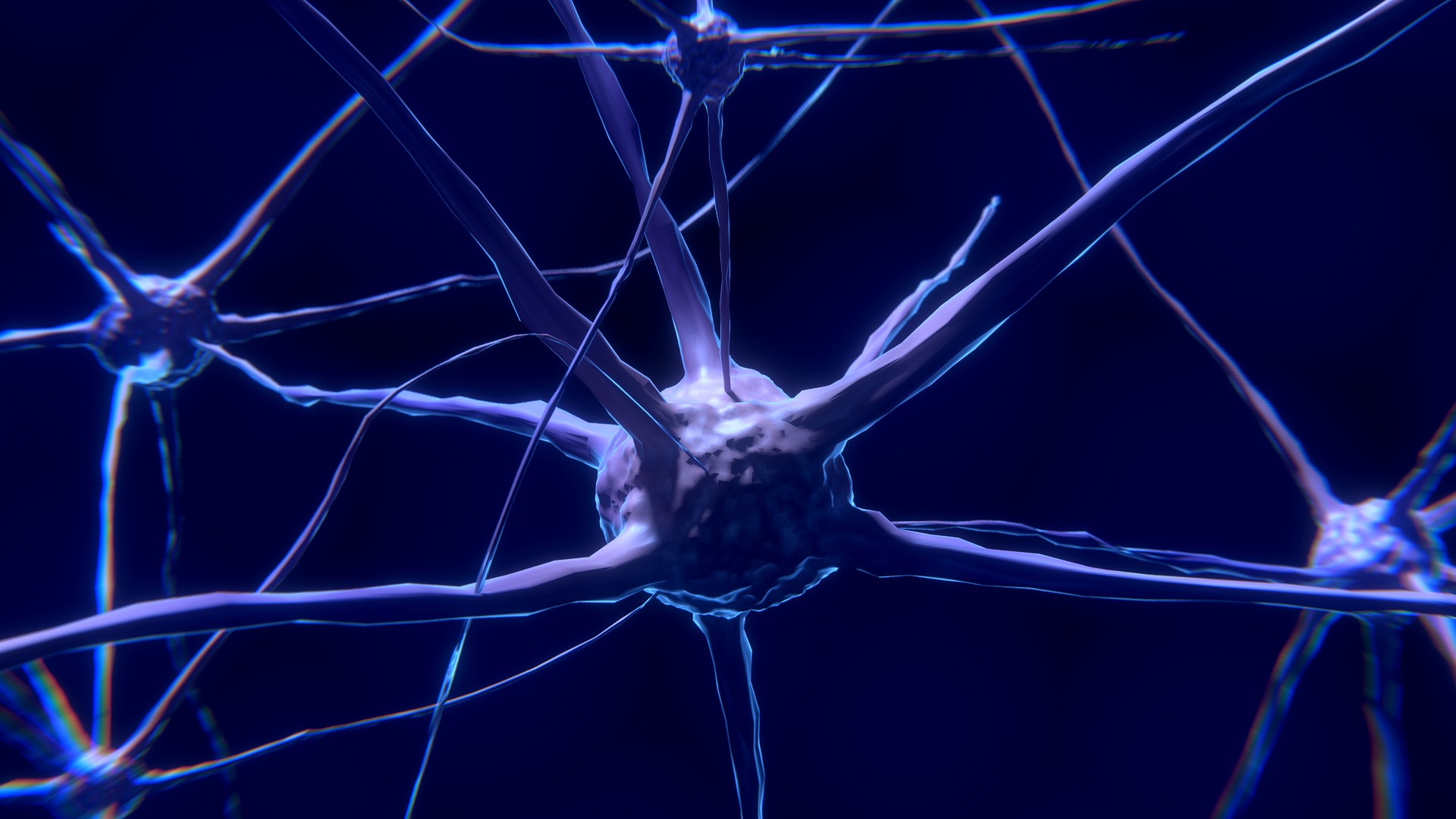
Pathways to the Future of AI
🥋 A recent TED talk by Jeff Dean reveals Google's plans for AI and Deep Learning in particular. Sidenote: Jeff Dean is the Chuck Norris of Google (google it!) and has helped create MapReduce, BigTable, and Tensorflow.
Featured image by: Colin Behrens from Pixabay
📺 Anyway, in the TED Talk, he does a quick history lesson on neural networks and the more recent history of the amazing things they have been able to do in the last decade. Then outlines three significant shortcomings of deep learning models:
- do one thing, and one thing only (narrow AI)
- handle a single modality of data (only images, text, speech, etc)
- they are dense, so the entire model is activated for every task
🤖 So it turns out, Google has a new AI Solution for all three problems called "Pathways". It can train a handful of general-purpose models that can do thousands or millions of things, deal with all modalities, and leverages sparse, high-capacity models, where relevant parts become activated as needed.
⚖️ Sound pretty cool and extremely powerful but... "with great power comes great responsibility". Dean continues the talk assuring the audience that Google is addressing Responsible AI concerns and upholding its own AI principles. Chris Anderson, the head of TED, then grilled him about Google's ethical commitments during the Q&A.
🧐 So I ask: Can Google be trusted to put ethics over profits? And does the neural architecture described by Jeff cover all the problems with AI? Or provide a roadmap for AGI?