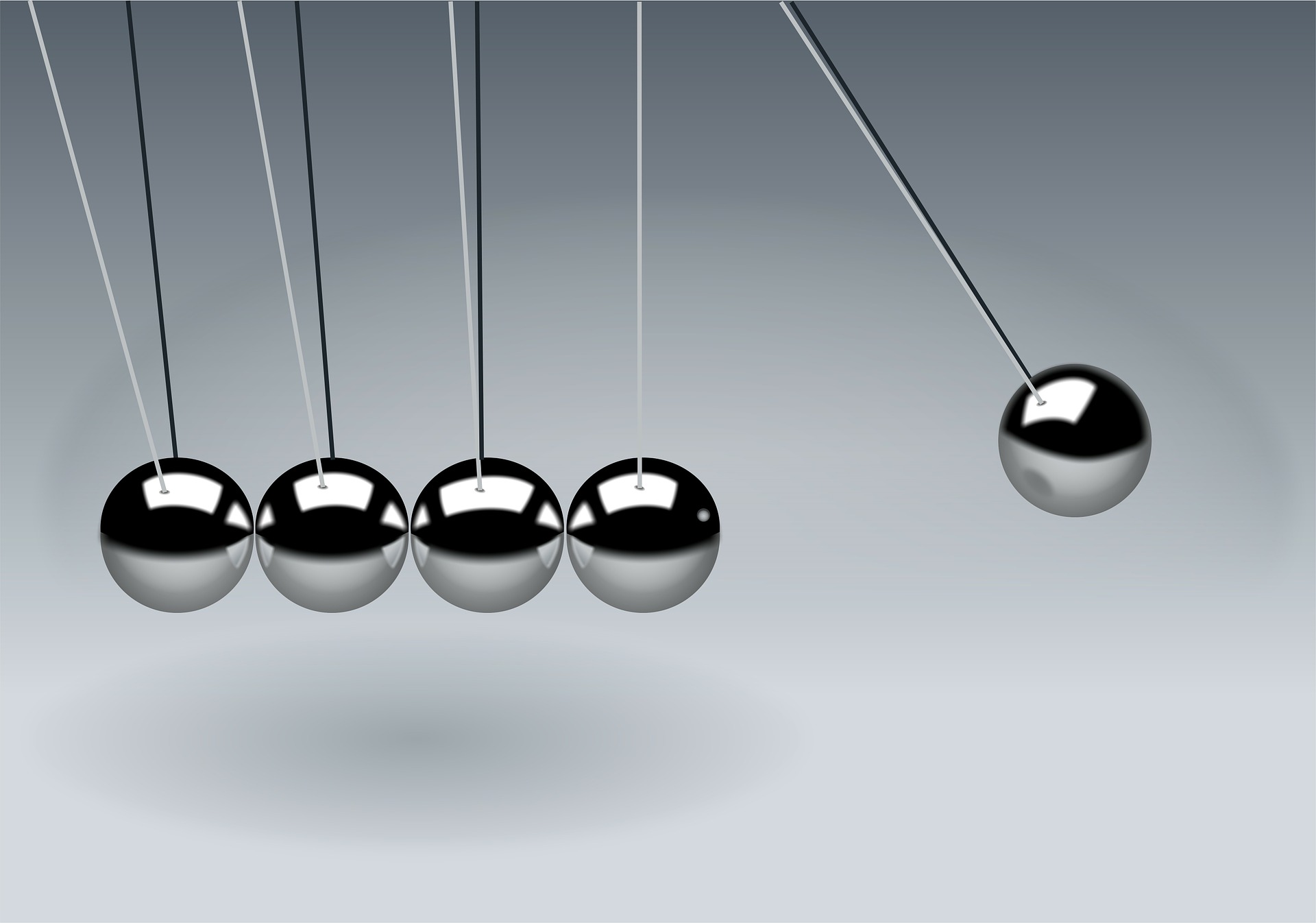
Causal Explanations of ML Models through Counterfactuals
We often hear "𝙘𝙤𝙧𝙧𝙚𝙡𝙖𝙩𝙞𝙤𝙣 𝙙𝙤𝙚𝙨 𝙣𝙤𝙩 𝙞𝙢𝙥𝙡𝙮 𝙘𝙖𝙪𝙨𝙖𝙩𝙞𝙤𝙣". And when working with data, it's easy to fall into this trap! Even aided by domain knowledge and complex models, it's often tough to disentangle both.
📈 I'm an advocate of 𝐈𝐧𝐭𝐞𝐫𝐩𝐫𝐞𝐭𝐚𝐛𝐥𝐞 𝐌𝐚𝐜𝐡𝐢𝐧𝐞 𝐋𝐞𝐚𝐫𝐧𝐢𝐧𝐠 (also known as XAI) because using approximations it can help understand models. However, I must accept that the most popular XAI methods rely on correlations, which is a significant limitation.
🔄 The solution to this problem is 𝐜𝐚𝐮𝐬𝐚𝐛𝐢𝐥𝐢𝐭𝐲 which yields a causal explanation rather than a correlation-based one. The authors of a recent paper (Leon Chou, Catarina Moreira, Peter Bruza, Chun Ouyang, and Joaquim Jorge), propose counterfactuals as a means to provide causability.
🤔 Counterfactuals are a good fit because they ask the question, "𝙬𝙝𝙖𝙩 𝙞𝙛?" which comes naturally to us humans and, given some properties, serves as a satisfactory causal explanation. There's a family of counterfactual methods that meet many of the properties. But, unfortunately, in recent years they have been overshadowed by other XAI methods.
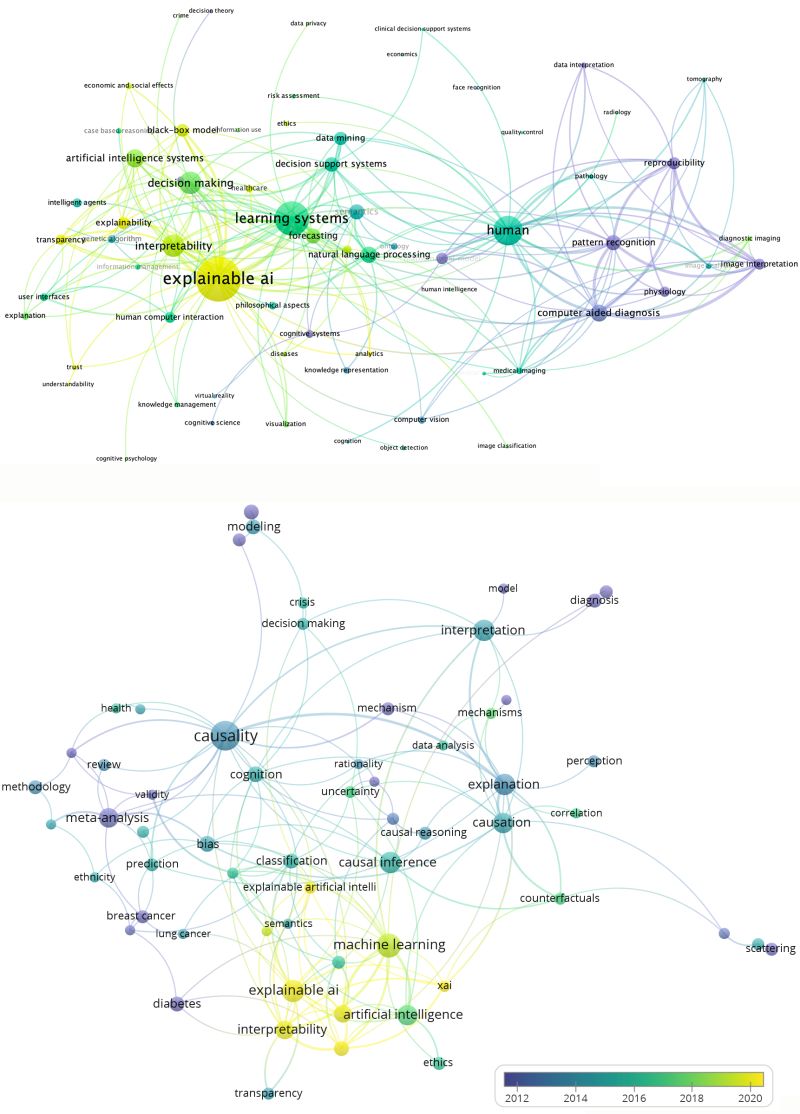
The authors of the paper performed a topic modeling and word co-occurrence analysis on academic research since 2012. It shows nodes for each keyword where size denotes frequency and color the most popular year. While it's good news that discussion has evolved from machine-centric topics such as pattern recognition to more human-centric such as XAI (see 1st figure), there are clear research gaps between causality and XAI - not to mention counterfactuals and causality (see 2nd figure). Check out their amazing paper for more details. Featured image by: Michal Jarmoluk from Pixabay