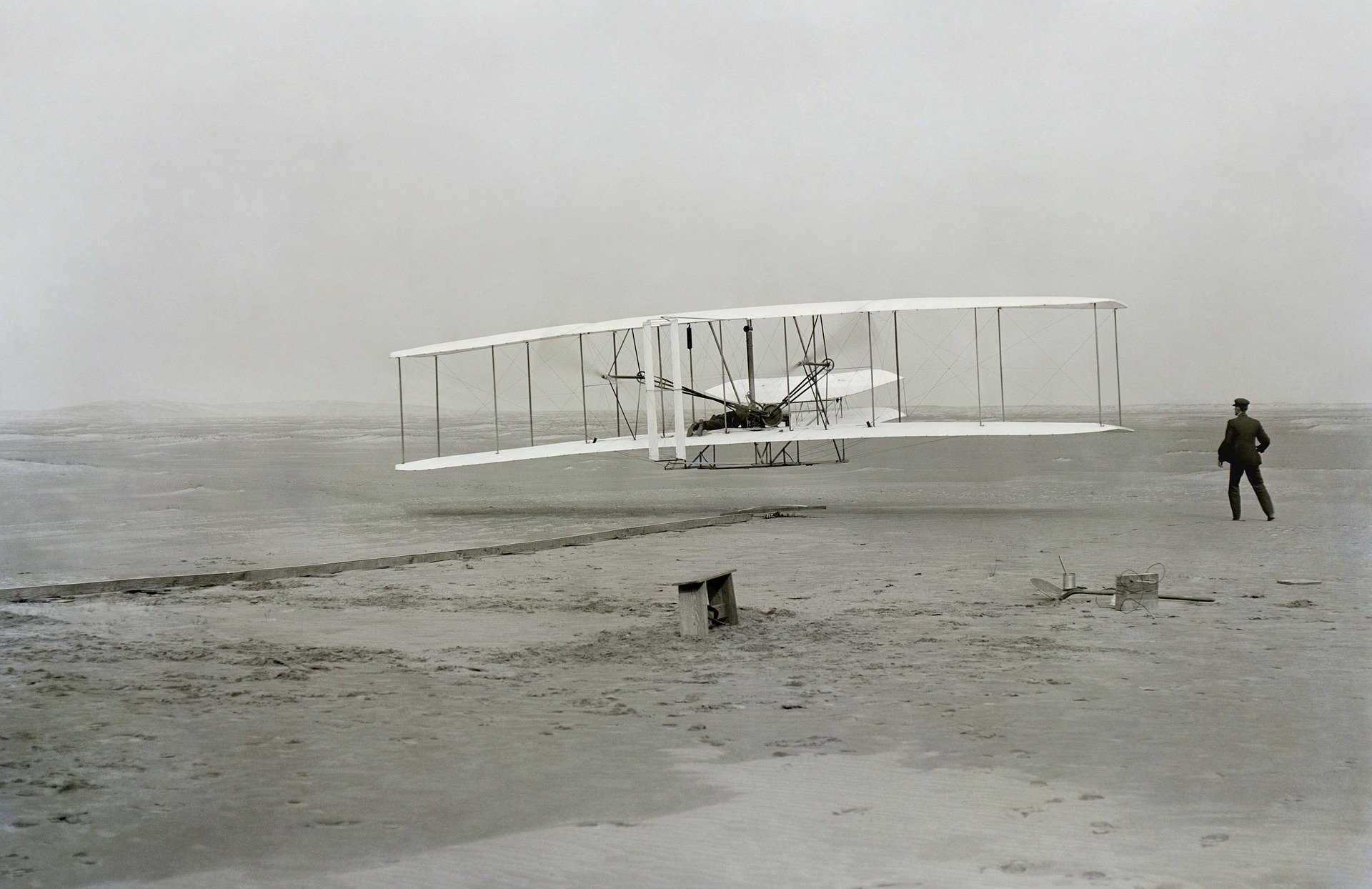
Why does Interpretable Machine Learning matter?
It's hard to tell from all the hype, but Artificial Intelligence 𝗶𝘀 𝗯𝗮𝗿𝗲𝗹𝘆 𝗶𝗻 𝗶𝗻𝗳𝗮𝗻𝗰𝘆 👶. But I'm hopeful that we can bring it into maturity.
☔ One of the most significant issues Machine Learning projects face is that models are ill-equipped to weather changing, adversarial, and 𝘂𝗻𝗲𝘅𝗽𝗲𝗰𝘁𝗲𝗱 𝗱𝗮𝘁𝗮 𝗰𝗼𝗻𝗱𝗶𝘁𝗶𝗼𝗻𝘀, much like planes facing storms and turbulence. But aircraft are robustly built and can overcome severe conditions both automatically and guided by experienced pilots. On the other hand, we know models must generalize well, but this proves to be an elusive property.
🎛️ Ever since I wrote my book, I've been asked many times why I'm passionate about 𝗜𝗻𝘁𝗲𝗿𝗽𝗿𝗲𝘁𝗮𝗯𝗹𝗲 𝗠𝗮𝗰𝗵𝗶𝗻𝗲 𝗟𝗲𝗮𝗿𝗻𝗶𝗻𝗴. I've responded that it's the instrument panel to pilot machine learning even in the worst conditions, from unfair to uncertain outcomes. So why wouldn't I prefer to have a complete instrument panel available? But, on the other hand, using predictive performance alone is like piloting with a single instrument!
Featured image by: WikiImages from Pixabay
✈️ Currently, flying is the safest mode of transportation. But for A.I., there is still a long way to go. For starters, we will need better no-code AutoML with human-in-the-loop and Interpretable M.L. built-in — like cockpits for Machine Learning engineers. And methods that automatically audit and test models, much like commercial planes, undergo strict maintenance regimens. And given what I've seen currently being built by Auto ML, MLOps, and XAI startups and researchers, it seems like it's heading in this direction, so I have reasons to be hopeful that for most commercial use cases, A.I. someday will be the 𝘀𝗮𝗳𝗲𝘀𝘁 𝗺𝗼𝗱𝗲 𝗼𝗳 𝗱𝗲𝗰𝗶𝘀𝗶𝗼𝗻-𝗺𝗮𝗸𝗶𝗻𝗴!