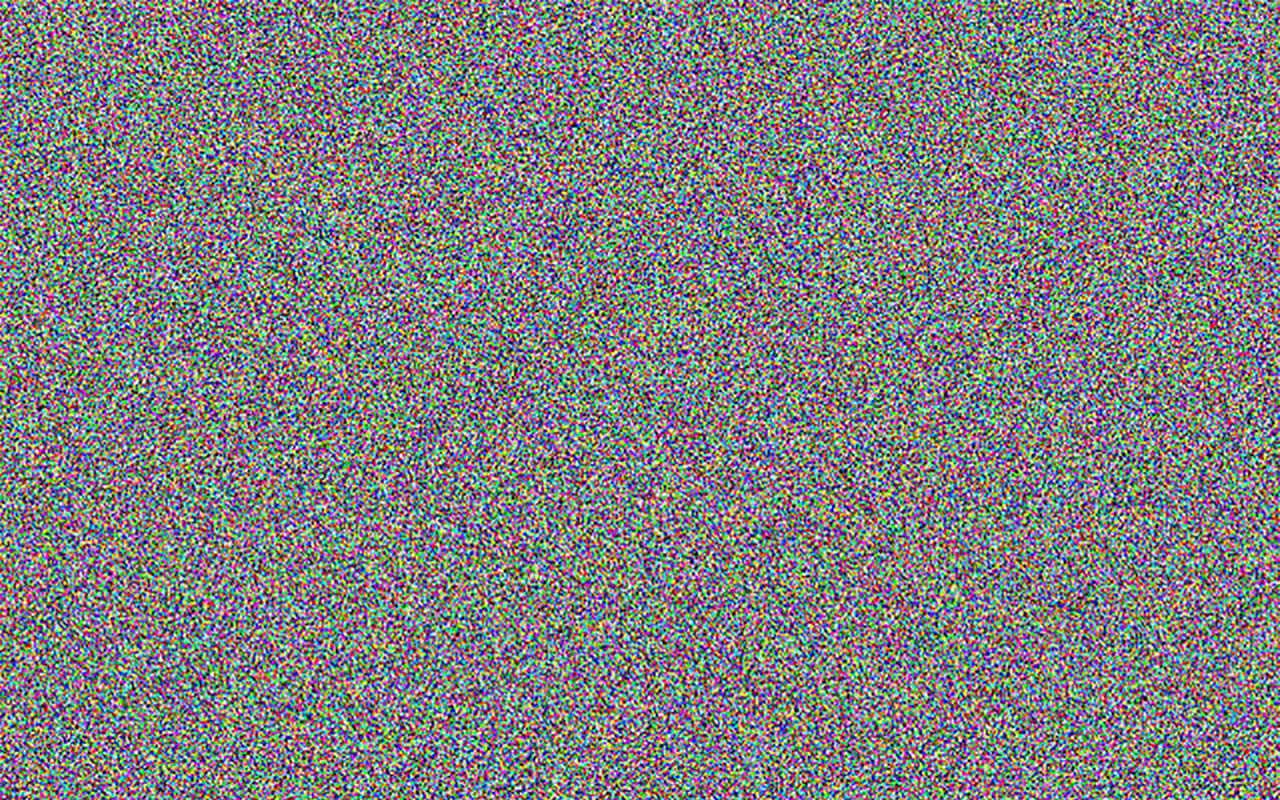
Book Review: Noise
When discussing human judgment and, by extension, algorithmic decisions, we are used to talking about ๐๐ข๐๐ฌ, but what about ๐ง๐จ๐ข๐ฌ๐?
Above picture by: Little, Brown Spark, Featured image by: Sophie Huiberts
๐ฏ Nobel Laureate แด แดษดษชแดส แดแดสษดแดแดแดษด and co-authors make a case for why we should pay close attention to it in their new book ๐๐๐๐ ๐: ๐ด ๐น๐๐๐ค ๐๐ ๐ป๐ข๐๐๐ ๐ฝ๐ข๐๐๐๐๐๐๐ก. It has some compelling stories to underpin how widespread the problem is in business and government with succinct illustrations. For instance, I love the target illustration and the error decompositions.
๐ข The book covers group dynamics such as information cascades, social pressure, group polarization as amplifiers of noise, and some cognitive #biases to boot. Lastly, it outlines noise mitigation strategies with decision hygiene, decision observers, and noise audits, which were BY FAR the biggest takeaways for me.
๐ However, if you are already familiar with the topic, the book will likely disappoint (at least a little). It can feel very repetitive and not getting into enough depth, and its entanglement with bias means it keeps referring to concepts covered in ๐โ๐๐๐๐๐๐ ๐น๐๐ ๐ก ๐๐๐ ๐๐๐๐ค, as it was some long-lost final chapter. I still enjoyed it, regardless.
Have you read it? Do you want to?