Predicting Rugby 7s Positions with Match-Play Statistics
The American Statistical Association for its 2019 Datafest, provided teams with a large dataset featuring one season’s worth of self-reported wellness data, training data, match results and match-play data for the Canadian Female Rugby team. The objective proposed by the organizers was simply to derive some insights from it. In choosing how to analyze the data, we found that because there were so few match results, it wouldn’t be statistically sound to attempt to find correlations. Instead, we found that we could make very accurate predictions about how obedient players were about the positions they were supposed to play on a second-to-second basis.
How consistently professional rugby players, or any team sport players for that matter, stick to the positions determined by the coach can be the difference between winning and losing matches. We:
• Leveraged millions of geospatial time-series data points from the Canadian Female Rugby 7s team season-long match-play data to get summary statistics on a second per second basis.
• Implemented two unsupervised models to predict the player positions at any given moment during any given game. One was a decision tree based on speed, distance and acceleration distributions drawn from a Rugby-specific academic paper. The other was a k-means model with k=6 accounting for 5 player positions and the bench. These models yielded an accuracy of 73% and 82% respectively.
• Won “Best Use of External Data” at American Statistical Association (ASA) Datafest 2019 competition.
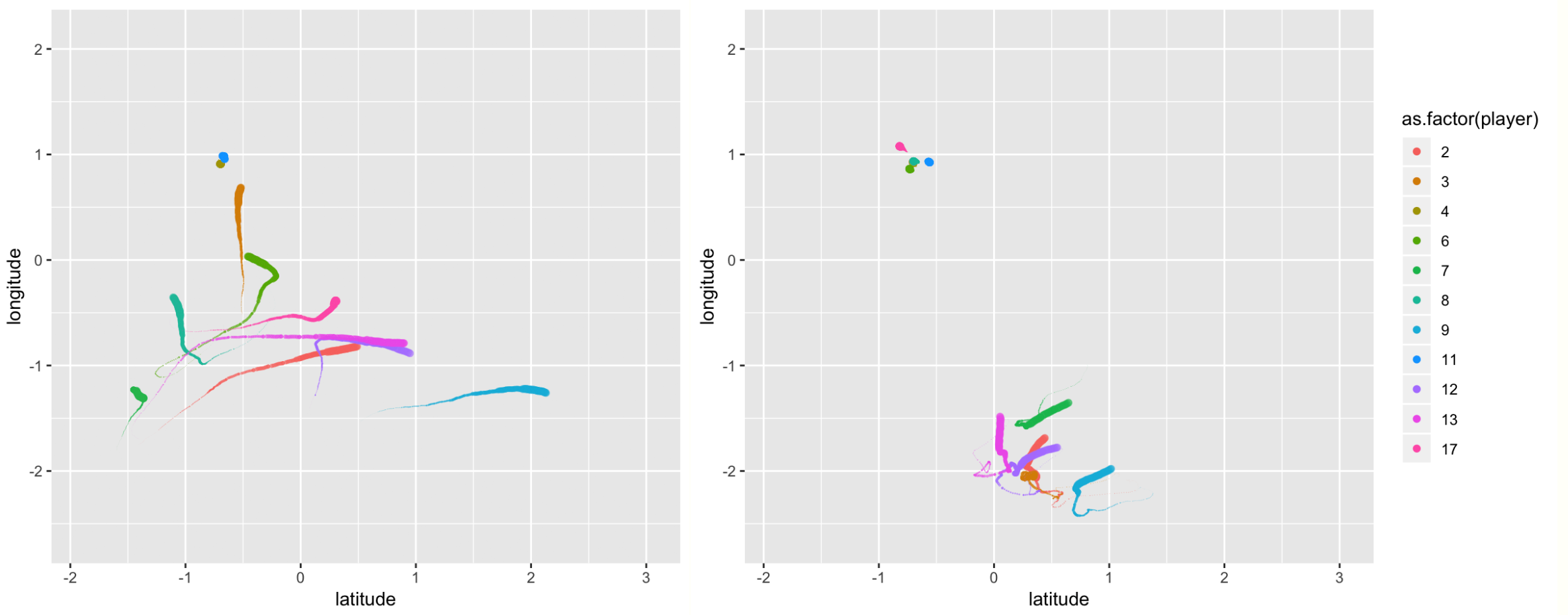
Summary
Using data from the Canadian female rugby team, my team and I won the American Statistical Association’s “Best Use of External Data” at their 2019 Datafest competition by predicting how well each player stuck the position they were assigned to play.